
[ad_1]
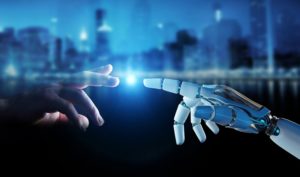
(Sdecoret/Shutterstock)
In the present day’s AI has advanced across the idea of recognition, which has undeniably been the linchpin of its progress. The power of AI to decipher textual content, speech, pictures, and video, executing intricate features primarily based on the understanding of the content material, has been a windfall not only for AI however for a myriad of industries.
Now in an period powered by generative AI (GenAI), fueled by massive language fashions (LLMs), new potentialities have impressed customers worldwide. On this novel panorama, AI fashions possess an unprecedented capability to reply to queries and requests with an unmatched depth and comprehensiveness. GenAI can craft full sentences and paragraphs with astonishing aptitude, and even delve into the realm of inventive expression, producing authentic art work and imagery.
As we enterprise additional into this uncharted frontier of AI, the anticipation builds, revealing the inescapable reality—the human contact stands as an indispensable power. Regardless of the exceptional capabilities of LLMs and GenAI like GPT-3, the human factor holds its irreplaceable significance.
The distinctive mix of understanding, empathy, and emotional intelligence discovered solely in people turns into the lifeblood that empowers LLMs and GenAI to traverse the divide between chilly automation and the heat of customized interactions.
Significance of Human Enter in Enhancing LLM
As generative AI evolves, so does the necessity for human enter.
We’re in an period of rediscovery in addition to a pendulum swing. The know-how is improbable however, as GenAI evolves, so does the necessity to merge AI with human mind. Whereas these information fashions have made important strides in producing high-quality content material, human intervention may help to make sure effectiveness, accuracy, and moral use. To unlock the total flexibility that an LLM has to supply, it must be expertly educated on generally hyper-specific datasets. That is completed by a method referred to as fine-tuning.
A method people can improve LLMs is thru information curation and refinement. LLMs are educated on huge quantities of knowledge, and consultants are essential of their capability to edit and filter information to take away biases, inaccuracies, or inappropriate content material. By rigorously choosing and making ready coaching datasets, people may help LLMs study from numerous and consultant sources, leading to unbiased efficiency, and assist make sure the AI mannequin’s recent content material is precisely labeled. People may also present experience and area information, permitting the generated content material to align with particular necessities or trade requirements.
The work doesn’t cease there, nevertheless. Human oversight can also be required to constantly monitor, evaluate and assess the generated content material, offering suggestions and corrections to refine the mannequin’s efficiency. This iterative suggestions loop between people and LLMs helps determine and rectify errors, bettering the mannequin’s accuracy and reliability over time.
Probably the most important methods people contribute is by guaranteeing the moral use of LLMs. By establishing tips and moral frameworks, people can be certain that LLM-generated content material adheres to societal norms, authorized necessities, and accountable AI practices. They will outline boundaries and constraints to forestall the technology of dangerous or deceptive info. Moreover, that is essential for industries, comparable to finance or healthcare, that are certain by strict compliance requirements.
From information assortment and curation to preprocessing, labeling, coaching, evaluating, refining, and deploying fine-tuning, human oversight to moral concerns, and analysis and improvement, people can contribute to enhancing the efficiency, accuracy, and accountable use of LLMs.
RLHF Requires Supervised High quality-Tuning
As soon as an AI mannequin is deployed and these enormous information units are being generated for labeling get bigger, the difficulty turns into tough to scale. On high of a fine-tuned mannequin’s capability to constantly enhance, the human layer maintains a gradual beat of reinforcement to make the mannequin smarter over time. That is the place reinforcement studying from human suggestions, or RLHF, is available in.
RLHF is a subfield of Reinforcement Studying (RL) that includes incorporating suggestions from human evaluators and a reward system to enhance the educational course of. By means of RLHF, firms can make the most of human suggestions for coaching their fashions to realize a greater understanding of their customers in order that they will reply to their customers’ wants leading to larger buyer satisfaction and engagement.
RLHF is offered in a number of methods, together with by rankings, ranking, and different strategies, to make sure that the outcomes of the LLM are optimizable in each relevant situation. RLHF requires sustained human effort and abilities and will be delivered by deploying a number of sources, together with area consultants, finish customers, crowdsourcing platforms, or third-party coaching information distributors.
RLHF elements embrace the next:
- Agent and Atmosphere – This introduces the fundamental elements of the RLHF framework, which includes an “agent” (an AI mannequin like GPT-3) interacting with an “setting” (the duty or drawback it’s attempting to resolve). This units the inspiration for understanding how the agent learns and improves by suggestions.
- Steady High quality-Tuning with Rewards and Penalties – This highlights the iterative studying course of in RLHF. The mannequin is constantly fine-tuned primarily based on the suggestions it receives within the type of rewards for proper actions and penalties for incorrect ones. This reinforcement mechanism helps the AI mannequin enhance its efficiency over time.
- Specialised Talent Units with Outsourcing Firms – This emphasizes the significance of getting specialised abilities and experience in producing correct and unbiased outputs utilizing RLHF.
It may be stated in impact that machines know nothing with out human enter. When information fashions are first being developed, human involvement is required at each stage to make an AI system competent, dependable, unbiased, and impactful. For instance, in healthcare, using such human consultants as board-certified docs and different educated clinicians, can make sure the output from the AI mannequin is factually correct.
By leveraging human experience and steerage, LLMs can proceed to evolve and turn out to be much more helpful instruments for producing high-quality, contextually related content material whereas guaranteeing moral and accountable AI practices.
The rise of generative AI is paving the best way for a brand new period of human-AI collaboration. As generative AI continues to advance, the collaboration between people and machines can be essential in harnessing the know-how’s potential for constructive influence. To make sure the thriving success of AI, industries putting a paramount emphasis on attaining a excessive stage of confidence in its outcomes can be crucial, ushering in an period the place people play a extra pivotal position than ever earlier than.
In regards to the creator: Rohan Agrawal is the CEO and Founding father of Cogito Tech, a supplier of AI coaching options that gives a human-in-the-loop workforce for pc imaginative and prescient, pure language processing, content material moderation, and information and doc processing.
Associated Objects:
Does the Human Contact + AI = The Way forward for Work?
Past the Moat: Highly effective Open-Supply AI Fashions Simply There for the Taking
Why LLMOps Is (In all probability) Actual
[ad_2]