
[ad_1]
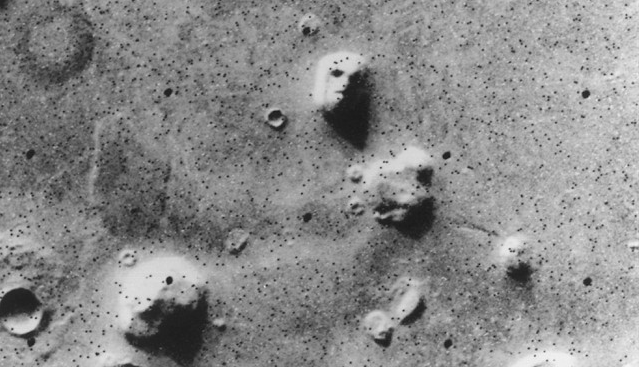
Supply: NASA/JPL
What do you see if you take a look at this photograph?
Most will see an image of a face. A face on a planet apart from Earth. Surprising, proper? An extraterrestrial object resembling a human face.
Under is similar geological characteristic, taken 21 years later. Nonetheless see a face, or do you now see a mountain?
This picture is without doubt one of the extra well-known examples of pareidolia. Pareidolia is the tendency for us to see patterns in nebulous photos or environment and attribute that means, on this case, a face the place there may be none. Our brains have this hard-wired into it due to the significance of faces in our evolutionary historical past.
You’ve got seen this elsewhere, in curtains, clothes, materials – see a face the place none exists. It’s a type of bias. It comes from a scarcity of knowledge from the enter. Lacking fragments of knowledge that we then, instinctually, fill in in order that we will “perceive” what we’re seeing.
Apparently, scientists viewing that first picture knew that information was lacking within the picture they obtained, so-called bit errors. They had been brought on by issues in transmission of the photographic information from Mars to Earth. These bit errors even precipitated a number of the visible parts that made the characteristic resemble a face.
These errors led to some amusing reactions, even entire books about buildings on Mars. And it was not the primary time our celestial neighbor had some wild claims about what would possibly exist on the floor. Within the latter half of the nineteenth century, when astronomers seemed by means of their telescopes, they noticed traces on the floor as canals.
This comes from a scarcity of knowledge, and the intrinsically human trait to seek out patterns on this planet, and past, to make sense of what we see.
The human mind does the identical. When studying, the reader doesn’t want the entire phrase within the right order. It’s attainable to learn lietrautre even with the letters within the flawed place if the primary and final are right. Don’t imagine me? Return and take a look at the phrase literature.
Why is that this necessary, and what does it should do with information bias and at present’s companies? Knowledge bias, whether or not it’s racial, ethnic, or stems from one other kind, exists in all lives. It may be hardwired from our nature, as above, or nurtured all through our lives. Because of this, it might probably make its means into expertise as nicely.
Knowledge bias can and does have an effect on how we perceive our enterprise or group and results in blind spots in lots of locations. Whether or not it’s analysis, security, or inhabitants information, lacking prison statistics, or within the worst-case deliberate omissions, information can hurt each the enterprise and finish customers. Injustices, employment, monetary inequalities, mischaracterization, medical malpractice, the record goes on.
Extra regarding is the dimensions of knowledge bias’s impression. With machine studying (ML), Synthetic Intelligence (AI), and different data-related applied sciences getting used at scale, with 100s of tens of millions of customers worldwide, the impression that errors within the information could cause are wide-ranging – as much as and together with, in uncommon circumstances, life-threatening.
Merely recognizing this doesn’t repair the problem. A latest examine performed by Perception Avenue confirmed that whereas 78% of enterprise and IT leaders imagine information bias will grow to be a much bigger downside, 77% of respondents acknowledge that they should do extra to know and handle bias. Solely a mere 13% are actively working to confront and finish information bias by means of an ongoing analysis course of.
Companies and organizations should act. They should meticulously assess their information units, determine bias in all its types, and reply appropriately to take away, replace, amend, or safe the information to realize probably the most complete understanding of their enterprise and the world they function in. Equally essential is the analysis of the expertise used. Knowledge platforms that may seize each structured and unstructured information whereas establishing strong guardrails to manipulate and defend the information are very important.
Moreover, utilizing expertise to produce human context, that means, and insights at machine scale is important. Exploring connections inside the information and making use of metadata to its supply can contribute to a extra correct illustration. These efforts ought to precede and comply with the information’s use in any system, particularly in ML and AI methods, the place the dimensions of bias amplification can result in inaccurate conclusions.
Companies have to belief the information they use, the data-driven choices made, and the actions taken to make sure the outcomes desired are right. Failure to deal with information bias adequately might lead to a lack of belief from end-users, doubtlessly affecting the success and sustainability of enterprises general.
Concerning the writer: Philip Miller is a buyer success supervisor for Progress and was named a Prime Influencer in Onalytica’s Who’s Who in Knowledge Administration. Exterior of labor, he’s a father to 2 daughters, a fan of canine and an avid learner, attempting to study one thing new daily.
Associated Gadgets:
Will Analytics Assist Scale back Our Biases or Amplify Them?
Three Methods Biased Knowledge Can Spoil Your ML Fashions
Watch out for Bias in Large Knowledge, Feds Warn
[ad_2]