
[ad_1]
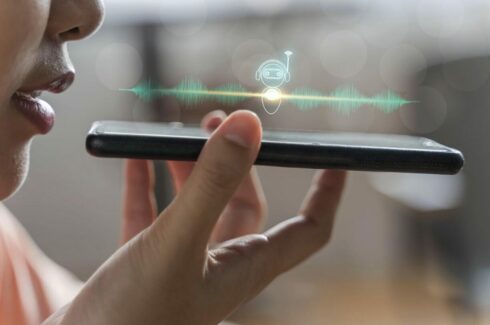
In late 2022, ChatGPT had its “iPhone second” and shortly turned the poster youngster of the Gen AI motion after going viral inside days of its launch. For LLMs’ subsequent wave, many technologists are eyeing the subsequent huge alternative: going small and hyper-local.
The core components driving this subsequent huge shift are acquainted ones: a greater buyer expertise tied to our expectation of instant gratification, and extra privateness and safety baked into consumer queries inside smaller, native networks such because the units we maintain in our palms or inside our automobiles and houses with no need to make the roundtrip to knowledge server farms within the cloud and again, with inevitable lag instances rising over time.
Whereas there’s some doubts on how shortly native LLMs may meet up with GPT-4’s capabilities resembling its 1.8 trillion parameters throughout 120 layers that run on a cluster of 128 GPUs, a number of the world’s greatest recognized tech innovators are engaged on bringing AI “to the sting” so new providers like sooner, clever voice assistants, localized laptop imaging to quickly produce picture and video results, and different varieties of client apps.
For instance, Meta and Qualcomm introduced in July they’ve teamed as much as run huge AI fashions on smartphones. The purpose is to allow Meta’s new giant language mannequin, Llama 2, to run on Qualcomm chips on telephones and PCs beginning in 2024. That guarantees new LLMs that may keep away from cloud’s knowledge facilities and their huge knowledge crunching and computing energy that’s each pricey and turning into a sustainability eye-sore for giant tech corporations as one of many budding AI’s business’s “soiled little secrets and techniques” within the wake of climate-change considerations and different pure sources required like water for cooling.
The challenges of Gen AI operating on the sting
Like the trail we’ve seen for years with many varieties of client know-how units, we’ll most actually see extra highly effective processors and reminiscence chips with smaller footprints pushed by innovators resembling Qualcomm. The {hardware} will preserve evolving following Moore’s Regulation. However within the software program facet, there’s been a number of analysis, improvement, and progress being made in how we are able to miniaturize and shrink down the neural networks to suit on smaller units resembling smartphones, tablets and computer systems.
Neural networks are fairly huge and heavy. They eat large quantities of reminiscence and wish a number of processing energy to execute as a result of they encompass many equations that contain multiplication of matrices and vectors that stretch out mathematically, related in some methods to how the human mind is designed to assume, think about, dream, and create.
There are two approaches which might be broadly used to scale back reminiscence and processing energy required to deploy neural networks on edge units: quantization and vectorization:
Quantization means to transform floating-point into fixed-point arithmetic, that is kind of like simplifying the calculations made. If in floating-point you carry out calculations with decimal numbers, with fixed-point you do them with integers. Utilizing these choices lets neural networks take up much less reminiscence, since floating-point numbers occupy 4 bytes and fixed-point numbers typically occupy two and even one byte.
Vectorization, in flip, intends to make use of particular processor directions to execute one operation over a number of knowledge without delay (through the use of Single Instruction A number of Knowledge – SIMD – directions). This accelerates the mathematical operations carried out by neural networks, as a result of it permits for additions and multiplications to be carried out with a number of pairs of numbers on the identical time.
Different approaches gaining floor for operating neural networks on edge units, embrace using Tensor Processor Items (TPUs) and Digital Sign Processors (DSPs) that are processors specialised in matrix operations and sign processing, respectively; and using Pruning and Low-Rank Factorization strategies, which includes analyzing and eradicating components of the community that don’t make related distinction to the end result.
Thus, it’s attainable to see that strategies to scale back and speed up neural networks may make it attainable to have Gen AI operating on edge units within the close to future.
The killer purposes that may very well be unleashed quickly
Smarter automations
By combining Gen AI operating regionally – on units or inside networks within the house, workplace or automotive – with varied IoT sensors related to them, it will likely be attainable to carry out knowledge fusion on the sting. For instance, there may very well be sensible sensors paired with units that may hear and perceive what’s occurring in your setting, scary an consciousness of context and enabling clever actions to occur on their very own – resembling routinely turning down music taking part in within the background throughout incoming calls, turning on the AC or warmth if it turns into too scorching or chilly, and different automations that may happen and not using a consumer programming them.
Public security
From a public-safety perspective, there’s a number of potential to enhance what we have now at present by connecting an rising variety of sensors in our automobiles to sensors within the streets to allow them to intelligently talk and work together with us on native networks related to our units.
For instance, for an ambulance making an attempt to succeed in a hospital with a affected person who wants pressing care to outlive, a related clever community of units and sensors may automate site visitors lights and in-car alerts to make room for the ambulance to reach on time. This kind of related, sensible system may very well be tapped to “see” and alert folks if they’re too shut collectively within the case of a pandemic resembling COVID-19, or to grasp suspicious exercise caught on networked cameras and alert the police.
Telehealth
Utilizing the Apple Watch mannequin prolonged to LLMs that would monitor and supply preliminary recommendation for well being points, sensible sensors with Gen AI on the sting may make it simpler to establish potential well being points – from uncommon coronary heart charges, elevated temperature, or sudden falls with no restricted to no motion. Paired with video surveillance for many who are aged or sick at house, Gen AI on the sting may very well be used to ship out pressing alerts to members of the family and physicians, or present healthcare reminders to sufferers.
Stay occasions + sensible navigation
IoT networks paired with Gen AI on the edge has nice potential to enhance the expertise at reside occasions resembling concert events and sports activities in huge venues and stadiums. For these with out flooring seats, the mixture may allow them to select a selected angle by tapping right into a networked digicam to allow them to watch together with reside occasion from a selected angle and placement, and even re-watch a second or play immediately like you may at present utilizing a TiVo-like recording system paired along with your TV.
That very same networked intelligence within the palm of your hand may assist navigate giant venues – from stadiums to retail malls – to assist guests discover the place a selected service or product is obtainable inside that location just by asking for it.
Whereas these new improvements are a minimum of a couple of years out, there’s a sea change forward of us for useful new providers that may be rolled out as soon as the technical challenges of shrinking down LLMs to be used on native units and networks have been addressed. Primarily based on the added pace and increase in buyer expertise, and diminished considerations about privateness and safety of maintaining all of it native vs the cloud, there’s quite a bit to like.
[ad_2]