
[ad_1]
MIT researchers have developed a novel method for analyzing unlabeled audio and visible knowledge, enhancing machine studying fashions for speech recognition and object detection.
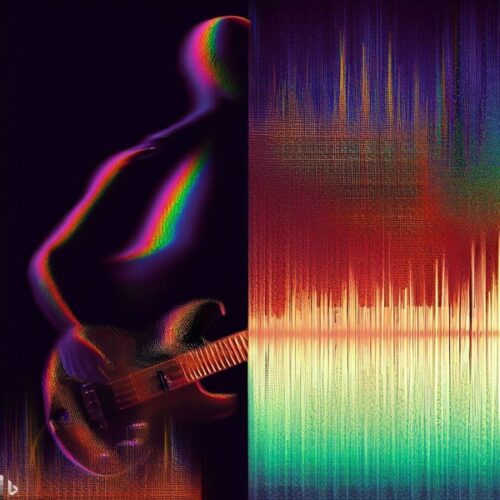
People usually purchase information via self-supervised studying because of inadequate supervision alerts. Self-supervised studying is the premise for an preliminary mannequin, leveraging unlabeled knowledge. Advantageous-tuning may be achieved via supervised studying or reinforcement studying for particular duties.
MIT and IBM Watson Synthetic Studying (AI) Lab researchers have developed a brand new methodology to research unlabeled audio and visible knowledge, enhancing machine studying fashions for speech recognition and object detection. The work merges self-supervised studying architectures, combining contrastive studying and masked knowledge modeling. It goals to scale machine-learning duties, similar to occasion classification, in numerous knowledge codecs with out annotation. This method mimics human understanding and notion. The contrastive audio-visual masked autoencoder (CAV-MAE), method, a neural community, learns latent representations from acoustic and visible knowledge.
A joint and coordinated method
CAV-MAE employs “studying by prediction” and “studying by comparability.” Masked knowledge modeling entails masking a portion of audio-visual inputs, that are then processed by separate encoders earlier than being reconstructed by a joint encoder/decoder. The mannequin is skilled based mostly on the distinction between the unique and reconstructed knowledge. Whereas this method could not totally seize video-audio associations, contrastive studying enhances it by leveraging them. Nonetheless, some modality-unique particulars, like video background, could must be recovered.
The researchers evaluated CAV-MAE, their methodology with out contrastive loss or a masked autoencoder, and different strategies on commonplace datasets. The duties included audio-visual retrieval and audio-visual occasion classification. Retrieval concerned discovering lacking audio/visible elements, whereas occasion classification recognized actions or sounds within the knowledge. Contrastive studying and masked knowledge modeling complement one another. CAV-MAE outperforms earlier methods by 2% for occasion classification, matching fashions with industry-level computation. It ranks equally to fashions with solely contrastive loss. Incorporating multi-modal knowledge in CAV-MAE improves single-modality illustration and audio-only occasion classification. Multi-modal data acts as a “delicate label” enhance, aiding duties like distinguishing between electrical and acoustic guitars.
Bringing self-supervised audio-visual studying into our world
The researchers take into account CAV-MAE a major development for purposes transitioning to multi-modality and audio-visual fusion. They envision its future use in motion recognition for sports activities, training, leisure, motor autos, and public security, with potential extensions to different modalities. Though at the moment restricted to audio-visual knowledge, the crew goals to focus on multimodal studying to imitate human talents in AI improvement and discover different modalities.
[ad_2]