
[ad_1]
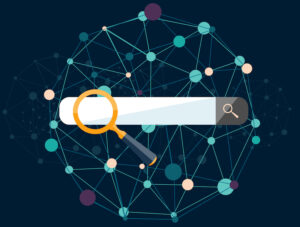
(theromb/Shutterstock)
Amazon Internet Providers is including vector search and vector embedding capabilities to 3 extra of its database companies, together with Amazon MemoryDB for Redis, Amazon DocumentDB, and Amazon DynamoDB, the corporate introduced yesterday at its re:Invent 2023 convention. It doesn’t appear to be the cloud big shall be including a devoted vector database to its choices.
Demand for vector databases is surging in the mean time, due to the explosion of curiosity in generative AI functions and enormous language fashions (LLMs). Vector databases are a crucial part of the rising GenAI stack as a result of they retailer the vector embeddings generated forward of time by LLMs, comparable to these provided in Amazon Bedrock. At runtime, the GenAI person enter in matched to a saved embedding by utilizing a nearest neighbor search algorithm within the database.
AWS added help for pgvector, a vector engine plug-in for PostgreSQL, to Amazon Relational Database Service (RDS), its PostgreSQL-compatible database providing, in Might. It added pgvector help to Amazon Aurora PostgreSQL-Suitable Version in July. With its bulletins this week at re:Invent, it’s including vector capabilities to its NoSQL database choices.
The addition of vector capabilities to Amazon MemoryDB for Redis will cater to prospects with the very best efficiency calls for for vector search, chatbots, and different generative AI functions, mentioned AWS VP of database, analytics, and machine studying Swami Sivasubramanian.
“Our prospects ask for an in-memory vector database that gives millisecond response time, even on the highest recall and the very best throughput,” he mentioned throughout his re:invent 2023 keynote on Wednesday. “That is actually troublesome to perform as a result of there’s an inherent tradeoff between velocity was as related of question outcomes and throughput.”
Amazon MemoryDB for Redis prospects will get “ultra-fast” vector search with excessive throughput and concurrency, Sivasubramanian mentioned. Even with hundreds of thousands of vectors saved, the service will ship single digit millisecond response time, “even when tens of 1000’s of queries per second at larger than 98% recall,” he mentioned. “This sort of throughput and latency is basically crucial to be used instances like fraud detection and actual time chat bots, the place each second counts.”
The corporate additionally introduced the overall availability of vector search capabilities in Amazon DocumentDB and Amazon DynamoDB, in addition to the GA of the beforehand introduced vector engine for Amazon OpenSearch Serverless.
Including vector search to DocumentDB permits prospects to retailer their vector embeddings proper subsequent to their JSON enterprise knowledge. That simplifies the GenAI stack, says Channy Yun, a principal developer advocate for AWS.
“With vector seek for Amazon DocumentDB, you possibly can successfully search the database based mostly on nuanced which means and context with out spending time and value to handle a separate vector database infrastructure,” Yun writes in a weblog. “You additionally profit from the absolutely managed, scalable, safe, and extremely out there JSON-based doc database that Amazon DocumentDB offers.” For extra info on the vector capabilities in DocumentDB, learn this weblog.
The corporate additionally introduced the GA of a vector engine in OpenSearch Serverless, the on-demand model of AWS’s Elasticsearch-compatible database. This beforehand introduced functionality will permit OpenSearch customers to make the most of similarity search together with different search strategies, like full textual content search and time-series evaluation, Yun writes.
“Now you can retailer, replace, and search billions of vector embeddings with 1000’s of dimensions in milliseconds,” Yun writes in a separate weblog. “The extremely performant similarity search functionality of vector engine allows generative AI-powered functions to ship correct and dependable outcomes with constant milliseconds-scale response instances.”
A zero-ETL connection between OpenSearch Serverless and Amazon DynamoDB, the corporate’s proprietary key-value retailer database, offers DynamoDB prospects entry to OpenSearch Serverless’s vector search capabilities, the corporate says.
Whereas it presents nearly each different database sort–together with a graph database, which additionally was enhanced with vector capabilities–AWS didn’t announce a devoted vector database, as some have been anticipating. It seems that AWS prospects choose vector capabilities in current databases relatively than a devoted vector database providing, in accordance with Sivasubramanian.
“They advised us they wish to use them of their current databases in order that they’ll remove the educational curve related when it comes to the educational a brand new programming paradigm, instruments, APIs,” Sivasubramanian mentioned throughout his re:Invent keynote on Wednesday. “In addition they really feel extra assured [in] current databases that they know the way it works, the way it scales, and its availability, and in addition evolves to satisfy the wants of vector databases.”
One other advantage of storing vector embeddings in an current database is that the functions will run quicker with much less knowledge overhead, Sivasubramanian added.
“There isn’t a knowledge sync or knowledge motion to fret about,” he mentioned. “For all of those causes, we’ve closely invested in including vector capabilities to a few of our hottest databases, together with Amazon Aurora, Amazon RDS, and OpenSearch companies.”
Associated Gadgets:
Amazon Launches AI Assistant, Amazon Q
5 AWS Predictions as re:Invent 2023 Kicks Off
Retool’s State of AI Report Highlights the Rise of Vector Databases
[ad_2]