
[ad_1]
Introduction
Welcome to the world of buyer suggestions evaluation, the place the unmined wealth of buyer opinions can form your online business’s triumph. In right this moment’s cutthroat competitors and with massive language fashions, comprehending buyer ideas is not a luxurious however a necessity. Buyer suggestions evaluation is each an artwork and a science—a methodical method to extracting actionable insights from numerous sources like surveys, evaluations, social media, and help interactions.
In right this moment’s digital realm, the place buyer suggestions flows extra abundantly than ever, companies relentlessly search methods to faucet into this wealth. This text introduces the fusion of AI and buyer suggestions evaluation, exploring how applied sciences like Pure Language Processing (NLP) and machine studying extract actionable insights. We uncover AI’s transformative potential in enhancing buyer satisfaction and driving enterprise triumph. Be a part of us on this enlightening journey as we discover the synergy between AI and optimizing buyer experiences.
Studying Targets
- AI Fundamentals: Grasp important AI ideas, together with NLP and enormous language fashions, and their relevance to buyer suggestions evaluation.
- AI Purposes: Discover AI’s sensible use in surveys, sentiment evaluation, suggestions categorization, and automatic responses, highlighting its effectivity.
- Actual-world Affect: Perceive AI’s real-world affect by way of numerous {industry} case research, showcasing its function in enhancing buyer experiences.
- Challenges and Ethics: Acknowledge AI challenges and moral issues in buyer suggestions evaluation, like knowledge high quality and privateness.
- Strategic AI Adoption: Learn to strategically make use of AI for aggressive benefit, enhancing decision-making, buyer focus, effectivity, intelligence, and innovation in suggestions evaluation.
This text was printed as part of the Knowledge Science Blogathon.
Understanding AI: A Temporary Overview
Synthetic Intelligence (AI) is a revolutionary know-how that seeks to copy human-like intelligence in machines and techniques. This transient overview supplies insights into AI’s core ideas and performance.
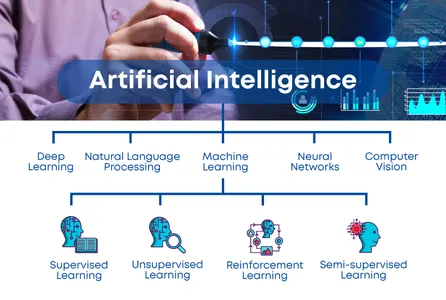
Mimicking Human Intelligence
At its coronary heart, AI endeavors to simulate human intelligence by enabling machines to be taught from knowledge, acknowledge patterns, make choices, and carry out duties that usually necessitate human cognition. It does this by way of a mixture of algorithms and knowledge.
The Position of Algorithms
Algorithms, or units of predefined guidelines and directions, type the muse of AI. These algorithms course of huge quantities of information, determine correlations, and use this info to make predictions or choices. Machine studying and deep studying are subsets of AI that concentrate on enhancing algorithmic efficiency by way of iterative studying from knowledge.
Knowledge as Gas
Knowledge serves because the lifeblood of AI. The extra high quality knowledge AI techniques have entry to, the higher their efficiency and accuracy. This knowledge can embody textual content, photographs, audio, or some other type of info that the AI system is designed to investigate or course of.
Kinds of AI
AI may be categorized into two most important sorts: Slim or Weak AI and Normal or Sturdy AI. Slim AI is designed for particular duties like language translation or picture recognition. On the similar time, Normal AI possesses human-like intelligence and may carry out a variety of duties akin to human cognition (though this degree of AI continues to be largely theoretical).
Purposes of AI
AI finds purposes in numerous domains, from healthcare and finance to customer support and autonomous automobiles. It powers chatbots, advice techniques, and enormous language fashions like GPT-3. These purposes leverage AI’s skill to investigate knowledge, perceive pure language, and make knowledgeable choices.
This transient overview affords a glimpse into the fascinating world of AI and its multifaceted performance. As we delve deeper into the article, we’ll see how AI is pivotal in enhancing buyer suggestions evaluation.
Leveraging Buyer Insights for Enchancment
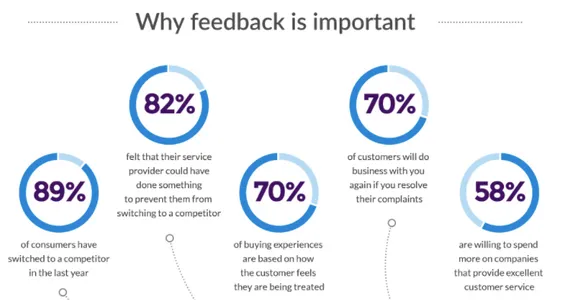
Buyer suggestions is a goldmine of insights that may considerably impression the expansion and enhancement of your online business. One of many basic steps in successfully analyzing buyer suggestions is to assemble it effectively, and right here’s how AI empowers this course of:
Maximizing the Energy of AI in Survey Creation
AI has reworked the best way companies accumulate buyer suggestions by way of superior survey instruments. AI-powered surveys leverage applied sciences like Pure Language Processing (NLP) to know what clients say and the sentiment and context behind their responses. Instruments like SurveySparrow allow the creation of interactive, human-like conversations as surveys. These enhance response charges and seize extra genuine and actionable suggestions.
Using Social Media Insights
Dive deep into how AI can effectively monitor and analyze buyer opinions shared on social media platforms associated to your model. AI-powered social listening instruments monitor mentions, sentiments, and traits throughout numerous platforms. This proactive method lets you collect buyer suggestions in real-time, serving to you determine rising points and alternatives.
Actual-time Buyer Suggestions Assortment with AI Chatbots
Discover the growing reputation of AI-driven chatbots for amassing real-time buyer suggestions. When built-in into your web site or cellular app, these chatbots have interaction clients in interactive conversations. AI-powered chatbots not solely interpret buyer responses intelligently but in addition present instantaneous help. This ensures a seamless and environment friendly suggestions assortment course of whereas enhancing buyer engagement.
Leveraging Voice of Buyer (VoC) Analytics
Learn the way AI-powered Voice of Buyer (VoC) analytics platforms analyze buyer interactions throughout numerous touchpoints, comparable to name heart conversations, help tickets, and emails, utilizing AI algorithms. These platforms assist extract insights from unstructured knowledge, determine rising patterns, and make data-driven choices to boost the general buyer expertise.
Automated Suggestions Evaluation with AI Instruments
Delve into the world of AI-powered suggestions evaluation instruments, which make use of superior strategies comparable to sentiment evaluation, textual content categorization, and subject modeling to course of and analyze knowledge mechanically. By using these instruments, companies can uncover hidden patterns, determine key themes, and prioritize areas for enchancment primarily based on buyer sentiments and preferences. These AI instruments allow data-driven decision-making, enhancing the general buyer suggestions evaluation course of.
AI revolutionizes how companies accumulate and interpret buyer suggestions, empowering them to make knowledgeable choices and repeatedly enhancing their choices to fulfill and exceed buyer expectations.
The Position of Giant Language Fashions
Giant language fashions, comparable to these primarily based on transformer structure, play a pivotal function within the realm of Synthetic Intelligence (AI) and pure language understanding. These fashions are impressed by the human mind’s skill to grasp and generate language. At their core, massive language fashions are subtle neural networks that excel in processing and producing textual content material. Their function may be summarized as follows:
Pure Language Understanding
Giant language fashions are designed to know human language in all its nuances. They’ll decipher the textual content’s that means, sentiment, and context, making them invaluable for duties like sentiment evaluation, textual content classification, and named entity recognition. This functionality is key for buyer suggestions evaluation, permitting companies to gauge buyer sentiments precisely.
Textual content Era
These fashions will not be restricted to understanding textual content; they will additionally generate it. Whether or not it’s producing human-like responses for chatbots, summarizing prolonged paperwork, and even creating inventive content material, massive language fashions can automate textual content era duties with outstanding fluency and coherence.
Buyer Engagement
Giant language fashions can improve buyer engagement in buyer surveys and suggestions evaluation. They are often employed to create conversational surveys, chatbots, and automatic responses that mimic human interactions. This boosts response charges and ensures that clients really feel heard and understood.
Multilingual Help
Giant language fashions excel in a number of languages, making them versatile instruments for companies working in numerous markets. They’ll analyze and generate textual content in numerous languages, enabling world firms to investigate buyer suggestions throughout language limitations successfully.
Personalization
These fashions can personalize buyer interactions primarily based on particular person preferences and historic knowledge. By understanding and predicting buyer wants, massive language fashions can tailor surveys and responses to offer a extra customized expertise, resulting in larger buyer satisfaction and loyalty.
Giant language fashions are the cornerstone of AI-powered buyer suggestions evaluation. They convey unparalleled pure language understanding and era capabilities, making them indispensable for companies looking for to unlock the true potential of buyer suggestions knowledge.
Exploring AI’s Position: The Energy of Giant Language Fashions
Within the realm of buyer suggestions evaluation, the emergence of enormous language fashions represents a transformative leap in AI capabilities. Primarily based on subtle transformer architectures, these fashions are revolutionizing how companies extract insights and that means from the huge troves of buyer suggestions knowledge. The facility of enormous language fashions on this context may be aptly summarized as follows:
Unmatched Pure Language Understanding
Giant language fashions possess an unmatched skill to know human language. They’ll decipher the intricacies of textual content, discerning not simply phrases but in addition context, sentiment, and nuances. This prowess allows companies to deeply perceive buyer sentiments, preferences, and ache factors, thereby driving extra knowledgeable decision-making.
Automated Sentiment Evaluation
These fashions excel in sentiment evaluation, a essential element of buyer suggestions evaluation. Giant language fashions can classify feedback as constructive, detrimental, or impartial by mechanically assessing the emotional tone of buyer suggestions. This automated sentiment evaluation streamlines the method of sorting and categorizing buyer suggestions, offering actionable insights at scale.
Easy Categorization and Tagging
Giant language fashions can categorize and tag buyer suggestions effortlessly. They’ll determine recurring themes and matters inside suggestions knowledge, permitting companies to prepare and prioritize areas for enchancment. This categorization saves time and ensures that related suggestions receives immediate consideration.
Environment friendly Response Era
These fashions can generate automated responses to buyer suggestions, guaranteeing that clients obtain well timed acknowledgments and resolutions. By simulating human-like interactions, massive language fashions improve buyer satisfaction and engagement. Companies may also tailor responses to particular buyer issues, additional personalizing the suggestions evaluation course of.
Multilingual Proficiency
Giant language fashions are proficient in a number of languages, making them invaluable for world companies. They’ll analyze buyer suggestions in numerous languages, breaking down language limitations and permitting firms to achieve insights from a various buyer base.
Personalization and Suggestion
Leveraging historic knowledge, massive language fashions can personalize surveys and suggestions interactions. They’ll advocate particular actions primarily based on buyer suggestions, offering companies with actionable insights for enhancing services and products. Personalization enhances buyer experiences, fostering loyalty and belief.
In essence, the facility of enormous language fashions in buyer suggestions evaluation is reshaping how companies perceive, interpret, and act upon buyer suggestions. These AI-driven fashions empower organizations to harness the complete potential of buyer opinions, enabling them to boost merchandise, providers, and buyer satisfaction with precision and effectivity.
Sensible Purposes of AI in Buyer Suggestions Evaluation
Chatbots for Actual-Time Help
AI-powered chatbots are digital assistants that use pure language processing (NLP) and machine studying to work together with clients in actual time. These chatbots can reply queries, present info, and information customers by way of numerous processes. They’re accessible 24/7, which boosts customer support availability. Chatbots can deal with routine inquiries, releasing human brokers to concentrate on extra complicated points. Over time, they be taught from interactions, turning into more and more environment friendly at offering correct and useful responses.
Customized Suggestions
AI-driven advice engines analyze massive volumes of buyer knowledge, together with previous purchases, searching historical past, and habits on an internet site or app. By understanding particular person preferences, these engines generate customized services or products suggestions. For instance, e-commerce platforms like Amazon use AI to recommend merchandise to clients primarily based on their searching and buy historical past. This personalization enhances the client expertise and will increase the chance of extra purchases, cross-selling, and upselling.
Sentiment Evaluation for Suggestions
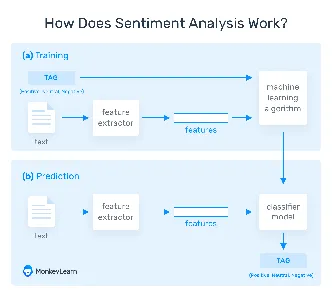
Sentiment evaluation, a subset of pure language processing, helps companies perceive how clients really feel about their services or products. AI algorithms analyze buyer suggestions, evaluations, and social media feedback to find out sentiment, comparable to constructive, detrimental, or impartial. This evaluation allows companies to gauge public notion precisely. For example, a restaurant can use sentiment evaluation to promptly determine and deal with detrimental evaluations, enhancing buyer satisfaction and popularity administration.
Voice Assistants and IVR Techniques
Voice-activated AI assistants, like Siri and Google Assistant, and Interactive Voice Response (IVR) techniques are utilized in customer support to allow voice-based interactions. Clients can carry out duties by talking to those techniques, comparable to checking account balances, making reservations, or getting product info. IVR techniques are generally utilized in name facilities to route calls and supply automated responses. They improve effectivity by lowering wait instances and permitting clients to entry info with out chatting with a dwell agent.
Predictive Analytics for Concern Decision
Predictive analytics makes use of historic knowledge and machine studying to determine patterns and traits. In customer support, it’s used to foretell potential points earlier than they happen. For example, a utility firm can use predictive analytics to anticipate tools failures and carry out preventive upkeep. This not solely reduces downtime but in addition minimizes buyer disruptions and complaints. Predictive analytics empowers companies to take proactive measures, enhancing customer support and operational effectivity.
These examples showcase how AI transforms customer support by automating duties, personalizing interactions, analyzing suggestions, and predicting and stopping points. Implementing AI applied sciences enhances the general buyer expertise and permits companies to proactively and effectively deal with buyer wants and issues.
Key AI Methods for Buyer Suggestions Evaluation
- Producing Survey Questions with AI: AI can help in creating efficient survey questions that seize worthwhile insights. Pure Language Processing (NLP) strategies allow the era of clear and related survey questions. AI algorithms analyze current survey knowledge, buyer suggestions, and industry-specific terminology to formulate questions that elicit actionable responses.
- AI-Pushed Sentiment Evaluation: Sentiment evaluation is a pivotal AI method for understanding buyer feelings and opinions. By using NLP and machine studying, AI can mechanically decide whether or not buyer suggestions is constructive, detrimental, or impartial. This evaluation supplies a complete view of buyer sentiment, serving to companies gauge satisfaction ranges and determine areas for enchancment.
- Categorizing and Tagging Suggestions: AI simplifies the method of categorizing and tagging buyer suggestions. NLP algorithms can determine recurring themes, matters, or points inside suggestions knowledge. This categorization streamlines knowledge group and permits companies to prioritize motion gadgets primarily based on widespread themes, guaranteeing that essential suggestions is addressed promptly.
- Producing Automated Responses: AI-driven techniques can generate automated responses to buyer suggestions. These responses are crafted primarily based on predefined templates and buyer suggestions evaluation. AI considers the context and sentiment of the suggestions to offer acceptable and well timed responses.
- Multilingual Help: AI applied sciences, significantly NLP fashions, are proficient in a number of languages. This multilingual functionality allows companies to investigate buyer suggestions in numerous languages. It breaks down language limitations and permits firms to achieve insights from a various buyer base, no matter language preferences.
These AI strategies empower companies to extract actionable insights from buyer suggestions effectively and successfully. By leveraging these strategies, organizations can higher perceive buyer sentiments, enhance services and products, and improve total buyer satisfaction.
Actual-World Affect: Case Research
On this part of our article, we are going to delve into cases the place AI has been deployed to investigate buyer suggestions, leading to tangible enterprise advantages. These case research are real-life examples of how AI applied sciences are making a distinction within the realm of buyer suggestions evaluation. Every case research will concentrate on a selected firm or group and the way they’ve harnessed AI to achieve worthwhile insights from buyer suggestions.
- Case 1: E-commerce Big – Customized Submit-Buy Surveys: An e-commerce firm makes use of AI to create customized post-purchase surveys. AI tailors survey inquiries to clients’ current purchases by analyzing buyer knowledge and behaviors. This personalization will increase survey engagement and helps collect worthwhile suggestions. The AI system additionally gauges buyer sentiment, resulting in improved product suggestions, larger buyer satisfaction, and elevated gross sales.
- Case 2: Hospitality Chain – Analyzing Buyer Critiques: A hospitality chain employs AI to investigate buyer evaluations from numerous on-line platforms. AI-driven sentiment evaluation categorizes evaluations as constructive, detrimental, or impartial and identifies key themes. This evaluation helps the chain spot traits in visitor experiences, deal with recurring points, and improve providers. The chain improves visitor satisfaction by performing on buyer suggestions, boosts its on-line popularity, and attracts extra guests.
- Case 3: Tech Help – Integrating Language Fashions into Chatbots: A tech help firm integrates massive language fashions into its chatbots. These fashions enhance chatbot interactions by understanding buyer queries in pure language, providing related troubleshooting steps, and producing automated responses. Chatbots can resolve widespread technical points and escalate complicated issues to human brokers. This leads to sooner and extra environment friendly help, lowered wait instances, and elevated buyer satisfaction. The mixing of language fashions additionally permits the corporate to scale its help operations cost-effectively.
Crucial Issues in Buyer Suggestions Evaluation
Within the realm of buyer suggestions evaluation, it’s essential to pay attention to numerous components that may impression the method. Understanding and addressing these points is crucial for efficient evaluation and harnessing AI’s full potential. Right here, we’ll discover some widespread challenges and issues that may come up when coping with buyer suggestions evaluation, together with methods to beat them.
- Knowledge High quality and Amount: Buyer suggestions knowledge can fluctuate in high quality, amount, and format. Incomplete, inconsistent, or biased knowledge can result in inaccurate insights. To deal with this problem, implement knowledge preprocessing strategies to scrub, manage, and construction the information. Use AI algorithms to fill in lacking info and guarantee knowledge accuracy.
- Moral and Authorized Points: Dealing with buyer knowledge raises moral and authorized issues, together with privateness, consent, transparency, and accountability. To navigate these challenges, guarantee strict compliance with knowledge safety laws and {industry} requirements. Prioritize transparency in knowledge dealing with and procure buyer consent when needed.
- Human Involvement and Oversight: Whereas AI can automate many features of research, human involvement is essential for outlining evaluation objectives, decoding outcomes, and guaranteeing moral use. To handle this consideration, foster collaboration between knowledge scientists, area specialists, and customer support groups. Set up clear roles for human oversight and decision-making within the suggestions evaluation course of.
- Mannequin Interpretability: Some AI fashions, significantly deep studying fashions, may be troublesome to interpret, making it difficult to know their decision-making processes. To beat this problem, use AI fashions that supply interpretability and transparency. Doc mannequin outputs and choices for accountability and understanding.
By contemplating these challenges and components, companies can navigate the complicated panorama of buyer suggestions evaluation extra successfully. AI applied sciences, with their superior capabilities, play a pivotal function in mitigating these challenges and enabling organizations to extract worthwhile insights from buyer suggestions whereas guaranteeing compliance, equity, and accuracy.
Incorporating AI for Strategic Benefit
- Enhancing Resolution-Making: AI empowers organizations to make data-driven choices swiftly and precisely. By analyzing huge datasets and extracting actionable insights, AI allows companies to reply promptly to market adjustments and client preferences.
- Buyer-Centric Method: AI-driven buyer insights and personalization are important for creating distinctive buyer experiences. Tailoring merchandise, providers, and advertising methods to particular person preferences can considerably enhance buyer satisfaction and loyalty.
- Streamlined Operations: Automation by way of AI enhances effectivity and reduces operational prices. Firms can optimize processes, decrease errors, and allocate sources extra successfully with AI-driven options.
- Aggressive Intelligence: AI allows companies to know opponents and market traits higher. Firms can determine rising alternatives and threats by way of superior analytics and predictive modeling, staying forward of the competitors.
- Innovation Catalyst: AI fosters innovation by automating repetitive duties, permitting workers to concentrate on inventive and strategic endeavors. This innovation can result in the event of groundbreaking services and products.
Our article will increase on these themes, exploring real-world examples of firms which have efficiently harnessed AI for a aggressive benefit. We may even delve into greatest practices for integrating AI into enterprise methods, emphasizing its function in driving progress, enhancing buyer relations, and streamlining operations. The insights gleaned from Jacobs and Firm’s article will inform our dialogue on how companies can successfully harness AI to take care of a aggressive edge in right this moment’s dynamic enterprise panorama.
Fundamental Sentiment Evaluation with VADER: Understanding Buyer Suggestions Sentiments
This Python script performs sentiment evaluation on an inventory of pattern buyer suggestions utilizing the VADER (Valence Conscious Dictionary and Sentiment Reasoner) sentiment evaluation instrument from the NLTK (Pure Language Toolkit) library.
Importing Libraries
The code begins by importing the mandatory libraries. It imports the NLTK library, particularly the SentimentIntensityAnalyzer class from the nltk.sentiment.vader module. VADER is a lexicon and rule-based sentiment evaluation instrument designed for textual content sentiment evaluation.
# Import needed libraries
import nltk
from nltk.sentiment.vader import SentimentIntensityAnalyzer
Pattern Buyer Suggestions
The code defines an inventory named suggestions containing three pattern buyer suggestions sentences. These sentences symbolize totally different sentiments: constructive, detrimental, and impartial.
# Pattern buyer suggestions
suggestions = [
"I love this product! It's amazing!",
"The customer service was terrible.",
"The quality of the product is excellent.",
]
Initializing the Sentiment Analyzer
The script initializes the sentiment analyzer by creating an occasion of the SentimentIntensityAnalyzer class and storing it within the variable sia.
# Initialize the sentiment analyzer
sia = SentimentIntensityAnalyzer()
Sentiment Evaluation Loop
The code then enters a loop to carry out sentiment evaluation on every sentence within the suggestions record. For every sentence, the polarity_scores methodology of the SentimentIntensityAnalyzer is used to calculate sentiment scores. The polarity_scores methodology returns a dictionary with 4 sentiment scores: constructive, detrimental, impartial, and compound.
# Carry out sentiment evaluation on every suggestions
for sentence in suggestions:
sentiment_scores = sia.polarity_scores(sentence)
# Decide sentiment primarily based on the compound rating
if sentiment_scores['compound'] >= 0.05:
sentiment="Constructive"
elif sentiment_scores['compound'] <= -0.05:
sentiment="Destructive"
else:
sentiment="Impartial"
Printing Outcomes
Lastly, the code prints every suggestions sentence and its decided sentiment (Constructive, Destructive, or Impartial).
# Print suggestions and sentiment
print(f"Suggestions: '{sentence}'")
print(f"Sentiment: {sentiment}n")
Output
The output of the code will show the sentiment of every suggestions sentence, whether or not it’s “Constructive,” “Destructive,” or “Impartial,” primarily based on the sentiment evaluation carried out by the VADER sentiment evaluation instrument. Right here’s what the output would possibly appear like for the supplied pattern suggestions sentences:
Suggestions: 'I really like this product! It is wonderful!'
Sentiment: Constructive
Suggestions: 'The customer support was horrible.'
Sentiment: Destructive
Suggestions: 'The standard of the product is great.'
Sentiment: Constructive
On this instance, the code analyzes three suggestions sentences and determines their sentiment. The primary and third sentences are categorised as “Constructive,” whereas the second sentence is assessed as “Destructive.”
This code is a primary instance of sentiment evaluation utilizing the VADER instrument, which is especially helpful for analyzing textual content knowledge specializing in social media content material and brief casual textual content. It’s vital to notice that VADER is a pre-trained lexicon-based mannequin and doesn’t require coaching on particular datasets. As an alternative, it depends on a predefined lexicon of phrases and their related sentiment scores to investigate textual content sentiment.
Conclusion
Understanding and responding to buyer suggestions is paramount for achievement within the dynamic enterprise panorama. This text has taken you on a journey by way of the world of buyer suggestions evaluation, showcasing the transformative affect of AI applied sciences.
From the foundational understanding of AI’s numerous strategies, comparable to Pure Language Processing (NLP), to the sensible purposes in survey era, sentiment evaluation, and automatic responses, we’ve unraveled how AI enhances effectivity, scales evaluation, and uncovers hidden insights inside huge datasets.
Actual-world case research have illuminated AI’s real-world impression, from customized surveys in e-commerce to improved buyer experiences within the hospitality {industry}. Nevertheless, embracing AI comes with challenges, together with knowledge high quality, ethics, and human involvement. But, with the strategic adoption of AI, companies can acquire a aggressive edge, enhancing decision-making, customer-centricity, operational effectivity, and innovation. This journey is a testomony to the transformative energy of AI in buyer suggestions evaluation, providing companies a compass to navigate the evolving panorama and thrive in a customer-centric world.
Key Takeaways
- Synthetic Intelligence (AI), together with NLP and enormous language fashions, transforms how companies extract insights from buyer suggestions.
- AI-driven surveys improve engagement by personalizing questions, leading to extra genuine and actionable suggestions.
- AI automates sentiment evaluation, streamlining suggestions categorization and offering actionable insights.
- Case research exhibit AI’s tangible advantages, from customized e-commerce surveys to improved hospitality experiences.
- AI adoption brings knowledge high quality and moral issues, necessitating cautious administration and human oversight.
- Strategic AI adoption enhances decision-making, customer-centricity, operational effectivity, and innovation in buyer suggestions evaluation.
Ceaselessly Requested Questions
A. A big language mannequin for customer support is an AI-powered system designed to know and generate human-like textual content responses to buyer queries and suggestions. It makes use of pure language processing (NLP) and deep studying strategies to help clients and enhance buyer help interactions.
A. The first goal of enormous language fashions in customer support is to boost the standard and effectivity of buyer interactions. These fashions can present instantaneous responses, reply incessantly requested questions, help in concern decision, and personalize buyer interactions, finally resulting in improved buyer satisfaction and operational effectivity.
A. One of the crucial outstanding massive language fashions for programming is OpenAI’s GPT-3.5. It may help in producing code snippets, providing programming-related explanations, and aiding builders in numerous programming duties. Nevertheless, the selection of the “greatest” mannequin could rely upon particular use instances and necessities.
A. Giant language fashions are evaluated primarily based on their skill to know and reply to buyer queries precisely, their velocity in responding, their language fluency, and their skill to adapt to context. The analysis additionally considers person suggestions and the mannequin’s efficiency in real-world customer support eventualities.
The media proven on this article is just not owned by Analytics Vidhya and is used on the Creator’s discretion.
Associated
[ad_2]