
[ad_1]
Introduction
Comprehending and unleashing the intricate affinities amongst variables within the expansive realm of statistics is integral.
All the pieces from data-driven decision-making to scientific discoveries to predictive modeling is dependent upon our potential to disentangle the hidden connections and patterns inside advanced datasets. Amongst numerous statistical requirements supporting this pursuit, covariance and correlation are essential, rendering insights into the independencies between variables.
Covariance and correlation are incessantly occurring variables in statistical evaluation, but folks usually misunderstand or use them interchangeably. The refined nuances differentiating these two requirements can profoundly influence our interpretation and utilization of statistical relationships.
Due to this fact, understanding the true nature of Covariance and correlation is paramount for any information fanatic or skilled striving to unveil the total potential of their information.
This blog- covariance vs correlation- will focus on the variations between these two statistical ideas and demystify their relationship.
Additionally, gas your information science profession by upgrading your expertise with Analytics Vidhya’s Study Swift for Knowledge Science course.
Covariance
It’s a statistical time period demonstrating a scientific affiliation between two random variables, the place the change within the different mirrors the change in a single variable.
Definition and Calculation of Covariance
Covariance implies whether or not the 2 variables are instantly or inversely proportional.
The covariance components determines information factors in a dataset from their common worth. For example, you possibly can compute the Covariance between two random variables, X and Y, utilizing the next components:

Within the above process,
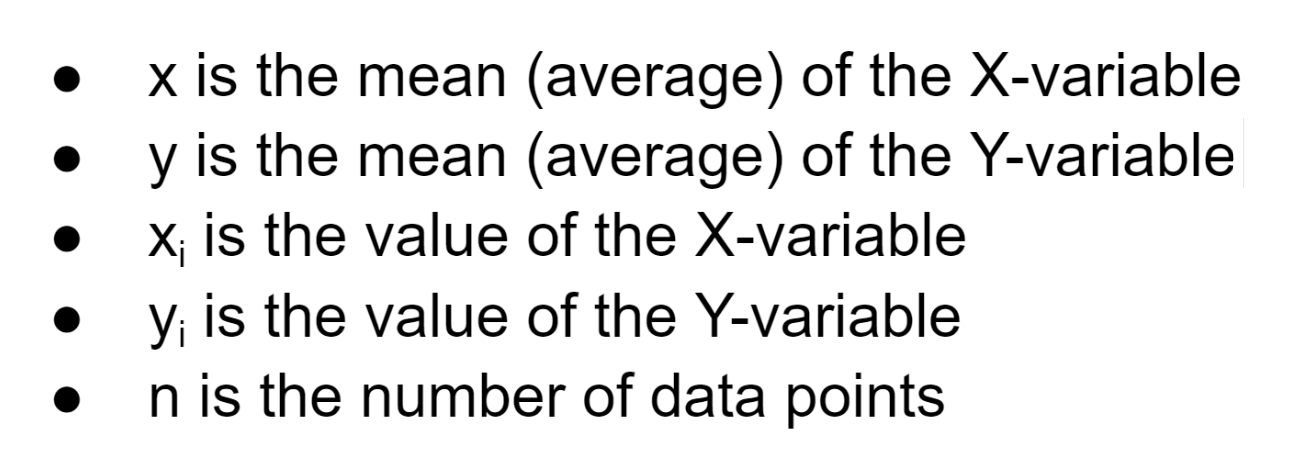
Deciphering Covariance Values
Covariance values point out the magnitude and route (optimistic or destructive) of the connection between variables. The covariance values vary from -∞ to +∞. The optimistic worth implies a optimistic relationship, whereas the destructive worth represents a destructive relationship.
Constructive, Adverse, and Zero Covariance
The upper the quantity, the extra reliant the connection between the variables. Let’s comprehend every variance kind individually:
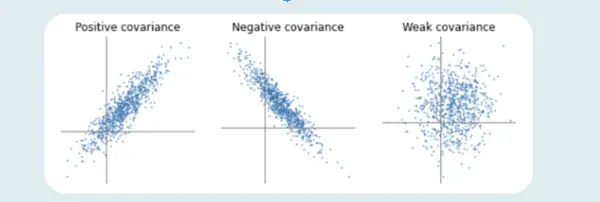
Constructive Covariance
If the connection between the 2 variables is a optimistic covariance, they’re progressing in the identical route. It represents a direct relationship between the variables. Therefore, the variables will behave equally.
The connection between the variables shall be optimistic Covariance provided that the values of 1 variable (smaller or extra important) are equal to the significance of one other variable.
Adverse Covariance
A destructive quantity represents destructive Covariance between two random variables. It implies that the variables will share an inverse relationship. In destructive Covariance, the variables transfer in the other way.
In distinction to the optimistic Covariance, the higher of 1 variable correspond to the smaller worth of one other variable and vice versa.
Zero Covariance
Zero Covariance signifies no relationship between two variables.
Significance of Covariance in Assessing Linear Relationship
Covariance is important in figuring out the linear relationship between variables. It suggests the route (destructive or optimistic) and magnitude of the connection between variables.
A better covariance worth signifies a powerful linear relationship between the variables, whereas a zero covariance suggests no ties.
Limitations and Concerns of Covariance
The scales of measurements affect the Covariance and are extremely affected by outliers. Covariance is restricted to measuring solely the linear relationships and doesn’t apprehend the route or power.
Furthermore, evaluating covariances throughout numerous datasets demand warning attributable to totally different variable ranges.
Correlation
In contrast to Covariance, correlation tells us the route and power of the connection between a number of variables. Correlation assesses the extent to which two or extra random variables progress in sequence.
Definition and Calculation of Correlation Coefficient
Correlation is a statistical idea figuring out the connection efficiency of two numerical variables. Whereas deducing the relation between variables, we conclude the change in a single variable that impacts a distinction in one other.
When an identical motion of one other variable reciprocates the development of 1 variable in some method or one other all through the research of two variables, the variables are correlated.
The components for calculating the correlation coefficient is as follows:
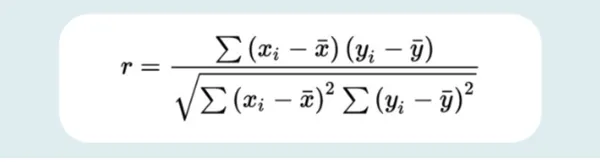
The place,
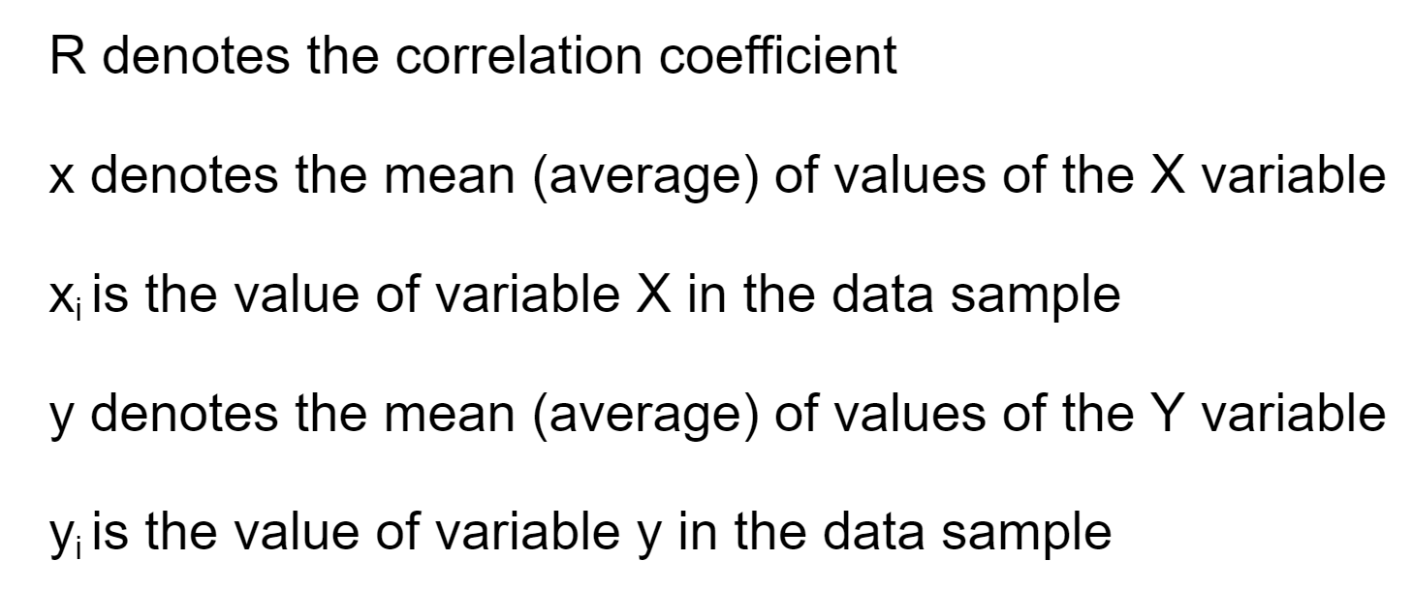
Deciphering Correlation Values
There are three varieties of correlation based mostly on numerous values. Adverse correlation, optimistic correlation, and no or zero correlation.
Constructive, Adverse, and Zero Correlation
If the variables are instantly proportional to 1 one other, the 2 variables are stated to carry a optimistic correlation. This means that if one variable’s worth rises, the opposite’s worth will exceed. A really perfect optimistic correlation possesses a worth of 1.
Right here’s what a optimistic correlation appears to be like like:
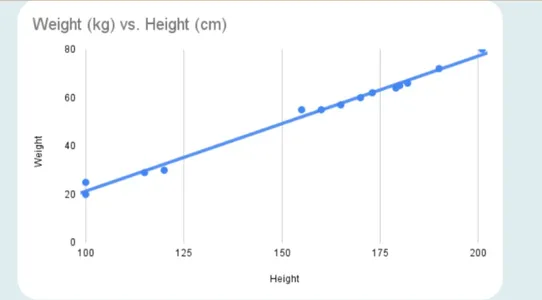
In a destructive correlation, one variable’s worth will increase whereas the second’s worth decreases. An ideal destructive correlation has a worth of -1.
The destructive correlation seems as follows:
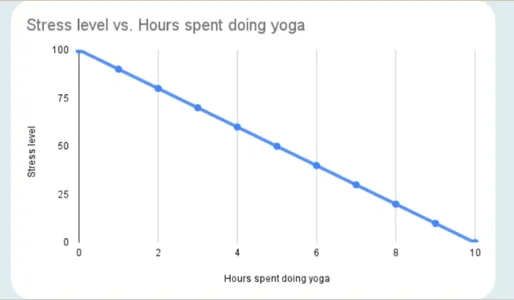
Identical to within the case of Covariance, a zero correlation means no relation between the variables. Due to this fact, whether or not one variable will increase or decreases gained’t have an effect on the opposite variable.
Power and Route of Correlation
Correlation assesses the route and power of a linear relationship between a number of variables. The correlation coefficient varies from -1 to 1, with values close to -1 or 1 implying a excessive affiliation (destructive or optimistic, respectively) and values close to 0 suggesting a weak or no correlation.
Pearson Correlation Coefficient and Its Properties
The Pearson correlation coefficient (r) measures the linear connection between two variables. The properties of the Pearson correlation coefficient embody the next:
- Power: The coefficient’s absolute worth signifies the connection’s power. The nearer the worth of the coefficient is to 1, the stronger the correlation between variables. Nonetheless, a worth nearer to 0 represents a weaker affiliation.
- Route: The coefficient’s signal denotes the route of the connection. If the worth is optimistic, there’s a optimistic correlation between the 2 variables, which signifies that if one variable rises, the opposite may also rise. If the worth is destructive, there’s a destructive correlation, which means that when one variable will increase, the opposite will fall.
- Vary: The coefficient’s content material varies from -1 to 1. The proper linear relationship is represented by a number of -1, the absence of a linear relationship is represented by 0, and an excellent linear relationship is denoted by a worth of 1.
- Independence: The Pearson correlation coefficient quantifies how linearly dependent two variables are however doesn’t suggest causality. There isn’t a assure {that a} robust correlation signifies a cause-and-effect connection.
- Linearity: The Pearson correlation coefficient solely assesses linear relationships between variables. The coefficient may very well be inadequate to explain non-linear connections absolutely.
- Sensitivity to Outliers: Outliers within the information would possibly affect the correlation coefficient’s worth, thereby boosting or deflating its measurement.
If you would like hands-on expertise engaged on Knowledge Science tasks, discover a complete program by Analytics Vidhya on Prime Knowledge Science Tasks for Analysts and Knowledge Scientists.
Different Varieties of Correlation Coefficients
Different correlation coefficients are:
- Spearman’s Rank Correlation: It’s a nonparametric indicator of rank correlation or the statistical dependency between the ranks of two variables. It evaluates how successfully a monotonic operate can seize the connection between two variables.
- Kendall Rank Correlation: A statistic determines the ordinal relationship between two measured values. It represents the similarity of the info orderings when ordered by every amount, which is a measure of rank correlation.
A picture of an anti-symmetric household of copulas’ Spearman rank correlation and Kendall’s tau are inherently odd parameter capabilities.
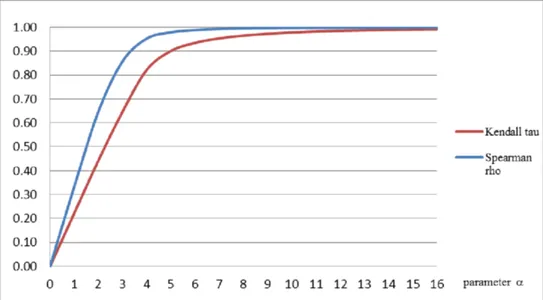
Benefits and Disadvantages of Covariance
Following are the benefits and downsides of Covariance:
Benefits
- Straightforward to Calculate: Calculating covariance doesn’t require any assumptions of the underlying information distribution. Therefore, it’s straightforward to calculate covariance with the components given above.
- Apprehends Relationship: Covariance gauges the extent of linear affiliation between variables, furnishing details about the connection’s magnitude and route (optimistic or destructive).
- Useful in Portfolio Evaluation: Covariance is usually employed in portfolio evaluation to judge the diversification benefits of integrating totally different property.
Disadvantages
- Restricted to Linear Relationships: Covariance solely gauges linear relationships between variables and doesn’t seize non-linear associations.
- Doesn’t Supply Relationship Magnitude: Covariance doesn’t provide a standardized estimation of the depth or power of the connection between variables.
- Scale Dependency: Covariance is affected by the variables’ measurement scales, making evaluating covariances throughout numerous datasets or variables with distinct models difficult.
Benefits and Disadvantages of Correlation
The benefits and downsides of correlation are as follows:
Benefits
- Figuring out Non-Linear Relationships: Whereas correlation primarily estimates linear relationships, it will possibly additionally reveal the presence of non-linear connections, particularly when utilizing different correlation requirements like Spearman’s rank correlation coefficient.
- Standardized Criterion: Correlation coefficients, such because the Pearson correlation coefficient, are standardized, various from -1 to 1. This permits for straightforward comparability and interpretation of the route and power of relationships throughout totally different datasets.
- Robustness to Outliers: Correlation coefficients are sometimes much less delicate to outliers than Covariance, delivering a stronger commonplace of the affiliation between variables.
- Scale Independencies: Correlation will not be affected by the measurement scales, making it handy for evaluating affinities between variables with distinct models or scales.
Disadvantages
- Pushed by Excessive Values: Excessive values can nonetheless have an effect on the correlation coefficient, regardless that it’s much less vulnerable to outliers than Covariance.
- Knowledge Necessities: Correlation assumes that the info is distributed in accordance with a bivariate regular distribution, which can not all the time be correct.
- Restricted to Bivariate Evaluation: As a result of correlation solely examines the connection between two variables concurrently, it will possibly solely seize easy multivariate correlations.
Similarities between Covariance and Correlation
Covariance vs correlation certain has a number of variations, however they’ve similarities too. A number of the similarities are as follows:
Indicators of the Relationship between Variables
Correlation and Covariance each gauge simply the linear relationship between variables. This means that if the correlation coefficient is zero, so will the Covariance. Even the change in location doesn’t have an effect on the correlation and covariance requirements.
The Measure of Linear Affiliation
Each Covariance and correlation are measures used to evaluate the connection between variables. They provide readability on how variables are linked to 1 one other.
Calculations Utilizing the Identical Variables
The calculation of Covariance and correlation each require the identical set of variables. They want
Covariance and correlation calculations contain the identical set of variables. They require paired observations of the variables of curiosity to find out their relationship.
Nonetheless, if it’s about selecting between Covariance and correlation to reckon the connection between variables, consultants all the time choose correlation over Covariance as a result of the change in scale doesn’t have an effect on correlation.
Variations between Covariance and Correlation
Whereas each of them are statistical phrases, Covariance and correlation differ from one another on numerous grounds.
Interpretation and Scale of Values
The change in scale modifications the worth of Covariance. A better quantity in Covariance means greater dependency. Deciphering Covariance is troublesome.
Quite the opposite, the correlation worth stays unaffected by the change in scale. The correlation coefficients vary from -1 to 1, which permits for a extra simple interpretation, in contrast to Covariance.
Relationship to the Items of Measurement
The variables’ measurement models have an effect on Covariance, making evaluating covariance values throughout numerous datasets or variables with totally different models difficult.
However, correlation coefficients don’t have any models and don’t depend on the models of measurement, permitting comparisons between variables with numerous models.
Standardization and Comparability Throughout Datasets
Since Covariance doesn’t have standardization, evaluating covariances throughout numerous datasets is difficult. Whereas correlation coefficients are standardized. Due to this fact, evaluating it instantly throughout variables, datasets, or contexts is straightforward.
Robustness to Outliers
Outlier vastly impacts the worth of Covariance. Therefore, it’s delicate to the presence of outliers. Quite the opposite, correlation coefficients provide a extra sturdy commonplace of the connection between variables, as correlation coefficients are much less vulnerable to outliers.
Utilization in Completely different Contexts and Functions
Covariance has purposes within the following:
- Biology — Molecular and Genetics to gauge particular DNAs.
- Estimating the invested quantity on totally different property in monetary markets.
- Accumulating information procured from oceanographic/astronomical research to conclude.
- Inspecting a dataset with logical implications of the principal factor.
- Finding out alerts obtained in several kinds.
However, the correlation has the next purposes:
- Employed in sample recognition
- Measures the connection between poverty and inhabitants
- Analyzes temperature rise throughout summer season v/s water consumption amongst relations
- Estimates the time vs. cash a buyer has spent on an internet e-commerce web site
- Compares the previous climate forecast stories to the present yr.
To summarize the variations, right here’s a desk you should look via:
Distinction Grounds | Covariance | Correlation |
Which means | Covariance means two variables instantly or inversely rely upon each other. | Two variables are stated to be in correlation if the change in a single impacts the opposite variable. |
Values | Lie between -infinity to +infinity | Values lie between -1 to 1 |
Unit | It’s a product of the unit of variables | It’s a unit-free measure |
Change in Scale | Even minor modifications in scale have an effect on Covariance | There gained’t be any change in correlation due to the dimensions |
Measure of | Correlation | The scaled model of Covariance |
Software | Market Analysis, Portfolio Evaluation, and Danger Help | Medical Analysis, Knowledge Evaluation, and Forecasting |
Use Circumstances and Examples
Given beneath are some sensible purposes and examples of Covariance vs Correlation:
Sensible Eventualities The place Covariance is Helpful
There are three sensible eventualities the place Covariance proves useful:
- Market Analysis: Covariance is employed in market analysis for analyzing the hyperlink between variables, comparable to gross sales income and promoting expenditure, to understand the affect of promoting endeavors on enterprise outcomes.
- Danger Evaluation: Covariance helps in danger administration and danger evaluation. For example, in insurance coverage, Covariance might help establish the affiliation between distinct variables (comparable to claims frequency, well being circumstances, and age) to evaluate potential losses and set applicable premiums.
- Portfolio Evaluation: Covariance has a profound software in finance for evaluating the connection between totally different asset returns inside a portfolio. A optimistic covariance signifies that the property will transfer in the identical route, whereas a destructive one signifies that they are going to go in reverse instructions. Such data is useful in portfolio diversification for managing danger.
Sensible Eventualities The place Correlation is Helpful
Some sensible circumstances of correlation embody forecasting, information evaluation, and medical analysis.
- Forecasting: Correlations support forecasters in figuring out the diploma to which they’ll predict one variable based mostly on the values of one other variable. For instance, correlation in gross sales forecasting could be useful in foretelling future gross sales based mostly on earlier gross sales data.
- Evaluation of Knowledge: Knowledge fanatics use correlation popularly to quantify and establish relationships between variables. For example, in social sciences, correlation might help decide the hyperlink between variables like schooling stage or revenue or between productiveness and job satisfaction.
- Medical Analysis: In medical analysis, correlations assist discover associations between variables, just like the correlation between lung most cancers and smoking or the correlation between the chance of cardiovascular ailments and the BMI (physique mass index).
Actual-World Examples and Functions of Covariance and Correlation
Whereas the purposes of Covariance and correlation have been described above, some real-world examples of the identical are as follows:
Covariances are used extensively in finance and present-day portfolio principle. For example, the Covariance between safety and the market are utilized within the calculation for one of many mannequin’s key variables, beta, within the capital asset pricing mannequin (CAPM), which determines the anticipated return on an asset.
Within the CAPM, beta quantifies an asset’s volatility, or systematic danger, in comparison with the entire market; it’s a beneficial metric that makes use of Covariance to find out an investor’s danger publicity specific to 1 funding.
Examples of Correlation embody the time spent working vs. the physique fats. The extra time an individual spends jogging, the much less physique fats they’ve. In different phrases, a destructive relationship exists between variable working time and variable physique fats. Physique fats lowers as working time rises.
One other occasion contains physique weight vs. top. The connection between a person’s measurement and weight is normally upbeat. In different phrases, those that are taller are inclined to weigh extra.
Factors to Contemplate Whereas Selecting Between Covariance and Correlation
Earlier than concluding or choosing Covariance or correlation, you should hold the next into consideration:
1. Concerns for the Analysis Query or Goal
Focus in your necessities. No matter you select should go along with the precise goal or analysis query. In case you intention to estimate the route and power of the linear affiliation between variables, then deciding on the correlation shall be clever. Nonetheless, when you want to measure the extent of a relationship with none interpretation, go for Covariance.
2. Nature of the Variables and Underlying Assumptions
The subsequent level to contemplate is the character of the variables you might be analyzing and the assumptions for every measure. The Correlation has no unit; it’s unitless and embraces a linear affiliation between variables. Nonetheless, Covariance focuses on the essential models and is delicate to the dimensions.
3. Availability of Knowledge and Measurement Scale
Don’t forget to evaluate the variable’s measurement scale and the accessible information. Whereas Covariance calls for paired observations of the variables, the correlation wants the same and bivariate normality assumption.
4. Significance of Standardized Interpretation or Comparability
You have to analyze the necessity for comparability or standardized interpretation throughout numerous variables or units of knowledge. If the comparability is crucial, the standardized vary of correlation varies from -1 to 1, permitting for easy comparability and interpretation.
5. Applicability within the Particular Evaluation or Discipline
You have to select a selected evaluation or subject of analysis. Whereas Covariance is relevant in market analysis, danger evaluation, and finance, correlation is employed in forecasting, information evaluation, and social sciences.
Conclusion
Understanding the variations between Covariance and correlation is important. Covariance measures the diploma of linear relationship, whereas correlation gives a standardized measurement that additionally considers the route and power of the connection.
Covariance vs correlation has a number of grounds for differentiation, comparable to standardization, interpretation, scale, sensitivity to outliers, and purposes in numerous phrases. Whereas they differ considerably, they do share some similarities as nicely. They’re indicators of the connection between variables and measures of linear affiliation.
Selecting an applicable measure is essential. It ought to be based mostly on particular necessities and the context of the evaluation. Covariance is useful in market analysis, danger evaluation, portfolio evaluation, and extra. In distinction, correlation is useful in medical analysis, forecasting, information evaluation, and different areas.
Why not do it with consultants if you wish to speed up your profession? Discover Analytics Vidhya’s Knowledge Science Hacks, Suggestions, and Methods course to sharpen your information science expertise and seize a rewarding profession as an information scientist.
Incessantly Requested Questions
A. A optimistic covariance implies that two variables are shifting in the identical route. It signifies a direct hyperlink between the variables. It means if the worth of 1 variable is extra glorious, the worth of one other variable may also be related.
A. The destructive Covariance means that the variables will share an inverse relationship. In destructive Covariance, the variables progress in the other way. This additionally signifies that if one worth has an above-average worth, the opposite could have a below-average worth.
A. The correlation coefficient of 1 means an excellent optimistic linear relationship between the variables. It means that if one variable will increase, one other one may also improve.
A. A correlation coefficient of 0 suggests no linear affiliation between the variables. It signifies that even when one variable modifications, one other will stay unaffected.
A. Covariance and correlation matrices provide insights into the relationships between numerous variables in an information set.
Associated
[ad_2]