
[ad_1]
Everybody’s speaking about machine studying lately, even us. We have now lined the fundamentals of what ML is and its historical past, however we wished to take a while to point out how individuals similar to you might be utilizing ML of their on daily basis lives. It could possibly look like a giant, scary science that solely large firms with numerous computing energy can deal with, however that could not be farther from the reality.
Customers of embedded electronics in every single place are utilizing ML to boost the performance of their work and tackle fixing extra advanced issues. Incorporating machine studying this fashion requires cautious consideration of useful resource constraints, energy effectivity, and mannequin measurement. Optimizing ML algorithms for embedded techniques and utilizing specialised {hardware} are widespread methods to make sure environment friendly operation inside these limitations. As machine studying methods proceed to advance, we are able to count on much more purposes of ML at a smaller scale!
Listed below are simply a number of the methods ML is being utilized in our subject, together with examples:
Sensor Knowledge Processing
Machine studying algorithms can course of knowledge from numerous sensors, corresponding to accelerometers, gyroscopes, cameras, and microphones, to extract significant data. For instance, ML can be utilized to acknowledge gestures, speech, or picture patterns, permitting for extra intuitive human-machine interplay and elevated utility alternative.
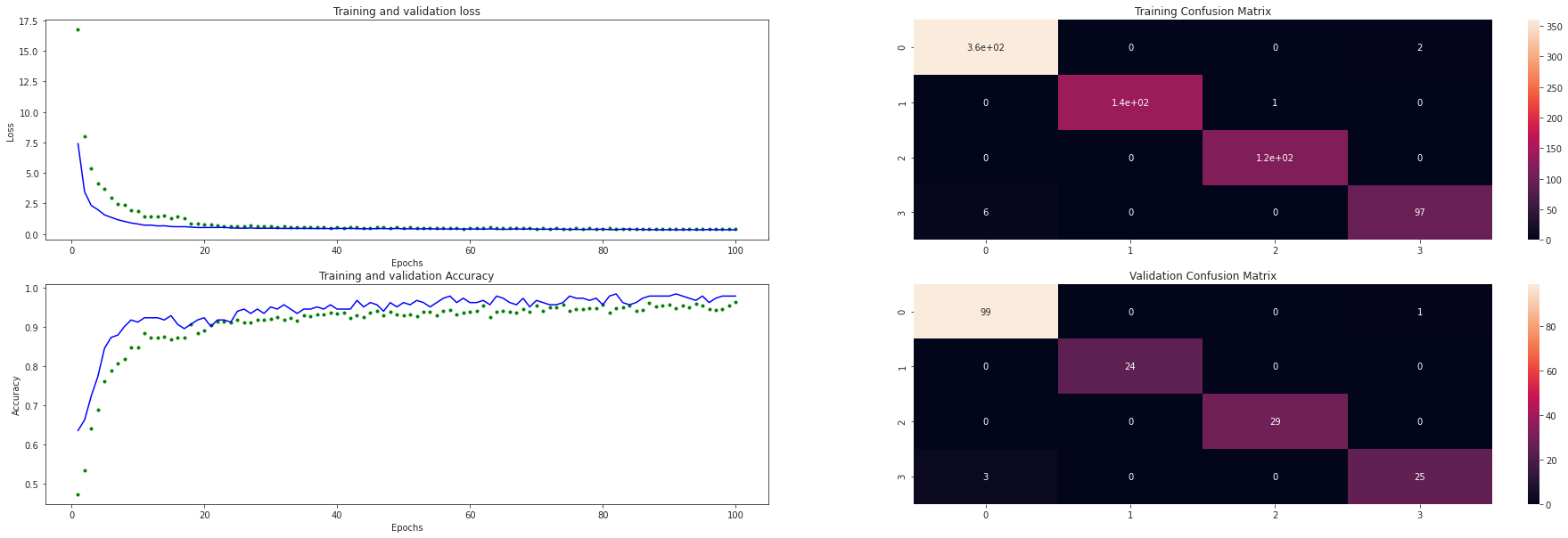
Anomaly Detection
Embedded techniques can use ML algorithms to detect anomalies or deviations from anticipated conduct in real-time sensor knowledge. That is beneficial for fault detection, occasion monitoring, and safety purposes.

Predictive Upkeep
ML fashions can analyze knowledge from sensors and predict when a chunk of kit or a system is prone to fail. This helps in scheduling upkeep duties effectively, decreasing downtime, and saving prices.
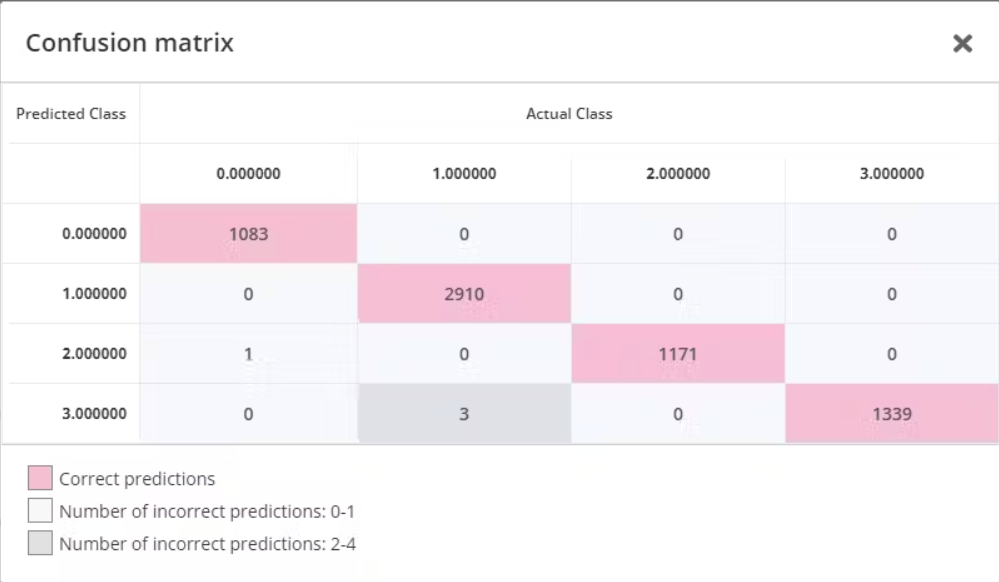
Pure Language Processing (NLP)
In purposes involving human-machine interplay, corresponding to chatbots or voice assistants, ML-powered NLP fashions can course of and reply to pure language queries and instructions.
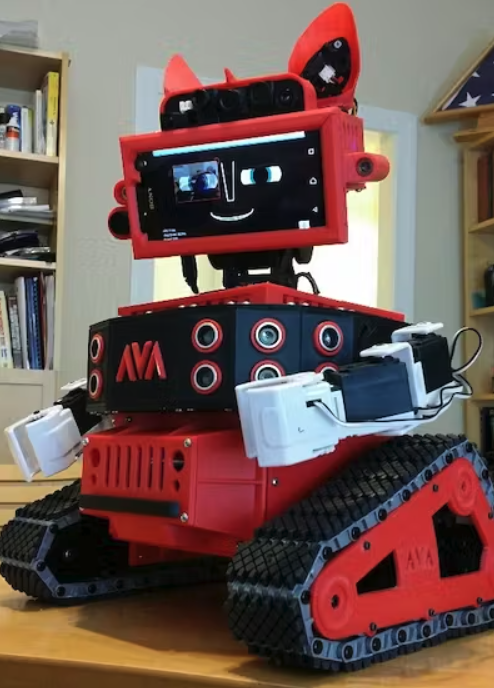
Pc Imaginative and prescient
Embedded gadgets with cameras can use machine studying for duties like object detection, face recognition, and picture classification. That is utilized in purposes starting from safety cameras to autonomous robots.
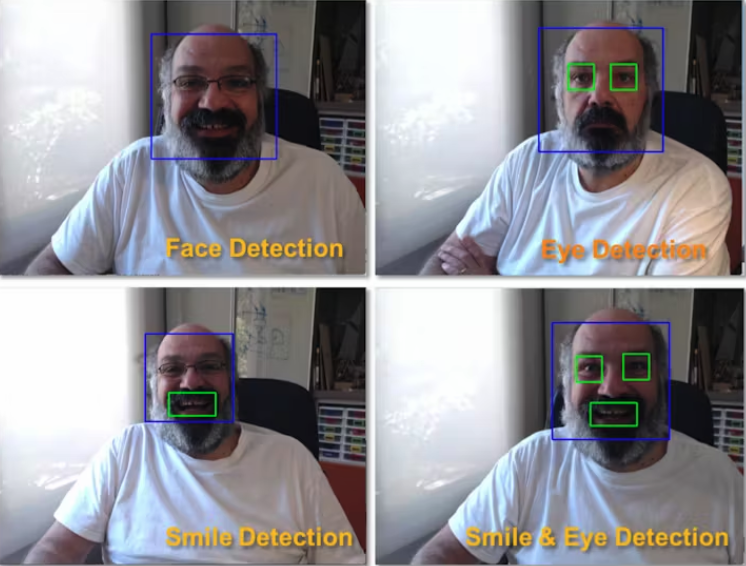
Vitality Optimization
Machine studying can optimize the power consumption of embedded techniques by predicting utilization patterns and adapting machine settings accordingly. That is significantly vital in battery-powered gadgets or when working with older or delicate energy techniques.
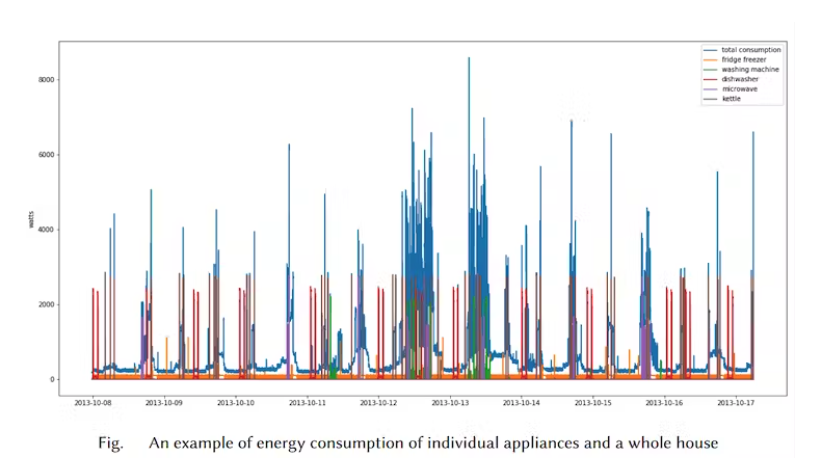
Autonomous Methods
Embedded ML can be utilized in autonomous automobiles, drones, and robotics to understand and reply to the surroundings. ML fashions assist in impediment avoidance, path planning, and navigation.
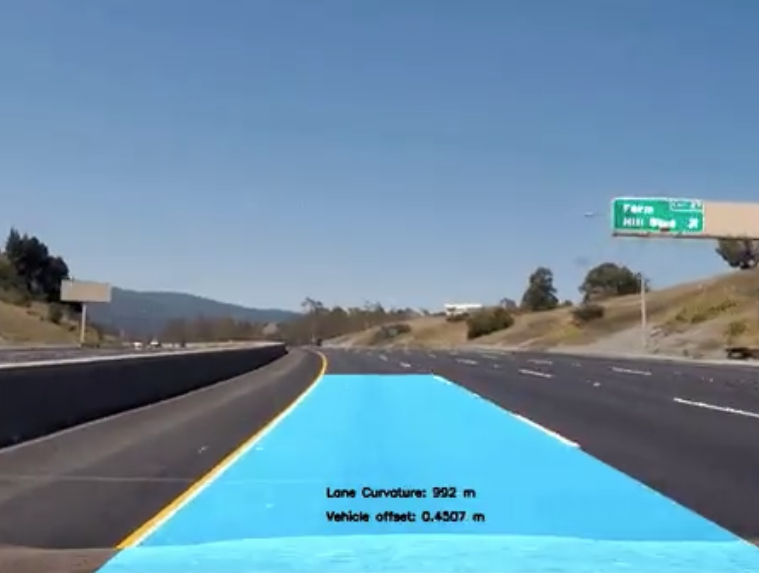
Medical Gadgets
In healthcare, embedded ML is used for duties like medical picture evaluation, affected person monitoring, and illness prediction. ML can help docs in diagnosing circumstances extra precisely.
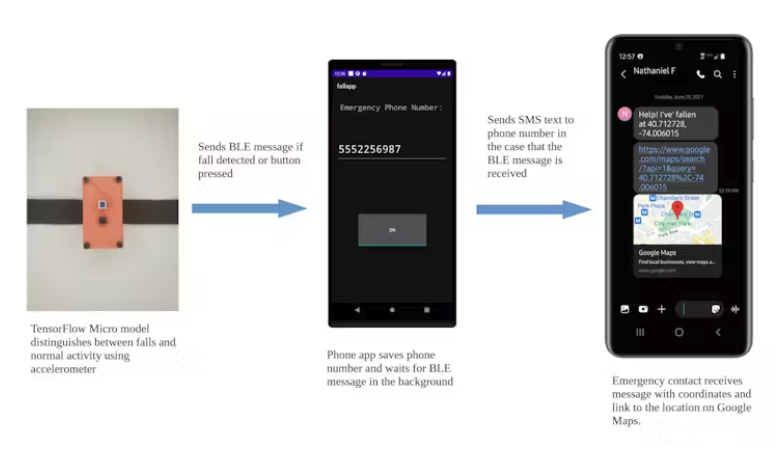
IoT Gadgets
Web of Issues (IoT) gadgets typically use ML to research sensor knowledge and make selections domestically. For instance, sensible thermostats can be taught person preferences and optimize heating or cooling.
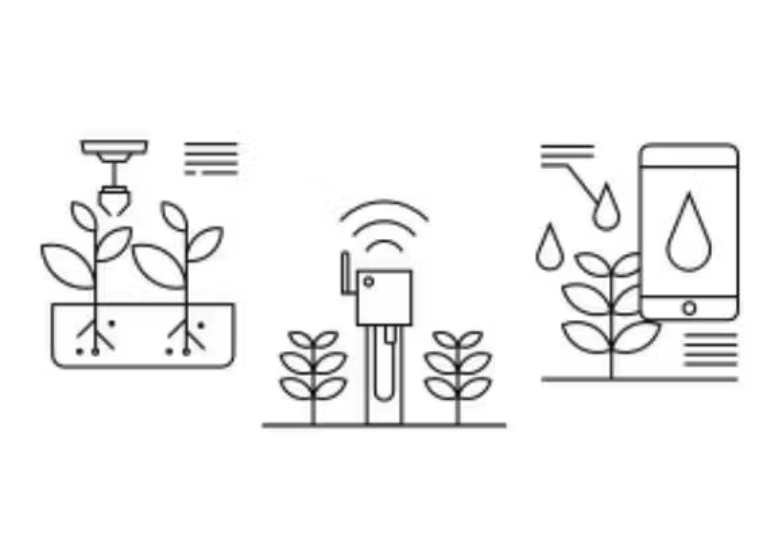
Safety
Embedded ML can improve safety techniques by figuring out and alerting to uncommon patterns or threats in real-time, enhancing the general security of linked gadgets and networks.
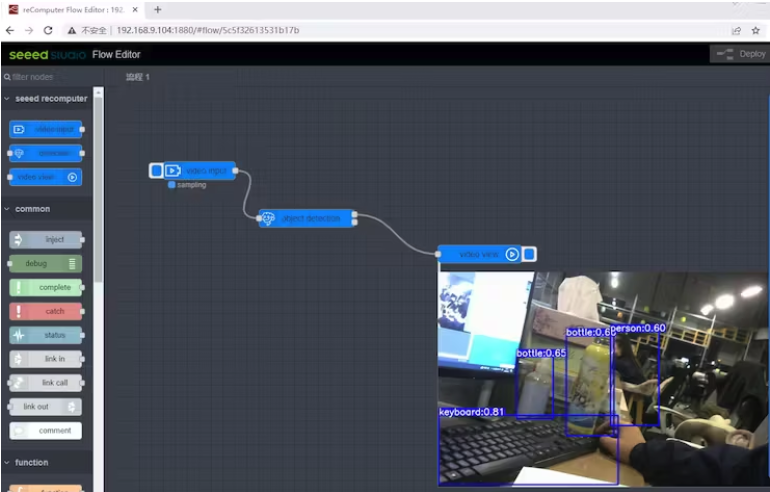
Additional Sources
Engaged on one thing with TinyML? Impressed by one of many above initiatives? Inform us about it within the feedback?
[ad_2]