
[ad_1]
Customers more and more anticipate to be engaged in a personalised method. Whether or not it’s an e-mail message selling merchandise to enhance a current buy, a web based banner advert asserting a sale on merchandise in a steadily browsed class or movies or articles aligned with expressed (or implied) pursuits, shoppers have demonstrated a choice for messaging that acknowledges their private wants and values.
Organizations that may meet this choice with focused content material have the alternative to generate increased revenues from client engagements, whereas people who can not run the danger of buyer defection in an more and more crowded and extra analytically refined retail panorama. Because of this, many organizations are making sizeable investments in personalization, regardless of financial uncertainty that’s slowing spend in different areas.
However the place to get began? As soon as a corporation has established processes for amassing and harmonizing buyer information from throughout varied contact factors, how may entrepreneurs use this information to offer higher content material alignment?
Propensity scoring stays one of the extensively adopted approaches for constructing focused advertising campaigns. The fundamental approach entails the coaching of a easy machine studying mannequin to foretell whether or not or not a buyer will buy an merchandise from inside a bigger group of merchandise inside a specified time frame. Entrepreneurs can use the estimated likelihood of a purchase order to determine not simply who to focus on with product-aligned campaigns however messages and affords to make use of to drive a desired end result.
Managing Quite a few, Overlapping Fashions Creates Complexity
The problem confronted by most organizations isn’t the event of a given propensity mannequin however the assist of the tens if not tons of of fashions required to cowl the assorted advertising campaigns inside which they’re participating. Let’s say a enterprise intends to run a marketing campaign targeted on grocery objects related to a mid-Summer season grilling occasion. The promotions group might outline a product group consisting of choose manufacturers of sizzling canines, chips, sodas and beer, and the advertising group would then have to have a mannequin created for that particular group. This marketing campaign might run concurrent with a number of different campaigns, every of which may have their very own, probably overlapping product teams and related fashions. Fairly quickly, the group finds itself juggling numerous fashions and workflows via which they’re employed to re-evaluate particular person clients’ receptiveness to product affords.
From the surface trying in, all this work is mirrored in a reasonably easy desk construction. Inside this construction, every buyer is assigned a rating for every product group (Determine 1). Utilizing these scores, the advertising group defines audiences/segments to affiliate with particular campaigns and content material.

However to the info scientists and information engineers chargeable for guaranteeing these scores are correct and updated, assembling this info requires the considerate coordination of three separate duties.
This Complexity Can Be Tackled via Three Duties
The primary of those duties is the derivation of function inputs. A few of these are merely attributes related to a consumer or product group that slowly change over time, however the overwhelming majority are metrics sometimes derived from transactional historical past. With every new transaction, beforehand derived metrics change into dated in order that information engineers are sometimes challenged to strike a steadiness between the price of recomputing these metrics and the influence of adjustments in these values on prediction accuracy.
Intently coupled to this primary activity is the duty of propensity re-estimation. As options are recomputed, these values are fed to beforehand skilled fashions to generate up to date scores (that are then recorded within the profile desk). The problem right here is to not solely generate the scores for all of the totally different households and lively fashions, however to maintain observe of which of the customarily tons of if not hundreds of function inputs are employed by a given mannequin.
Lastly, information scientists should think about how buyer conduct adjustments over time and periodically retrain every mannequin, permitting it to be taught new insights from the historic information that can assist it generate correct predictions within the interval forward.
Databricks Helps Coordinate These Duties
Maintaining with all these challenges whereas juggling so many various fashions can begin to really feel a bit overwhelming, however the information scientists and engineers tasked with managing this course of can simplify issues significantly by managing these duties as a part of two normal workflows and by profiting from key options within the Databricks platform meant to help them with these processes (Determine 2).
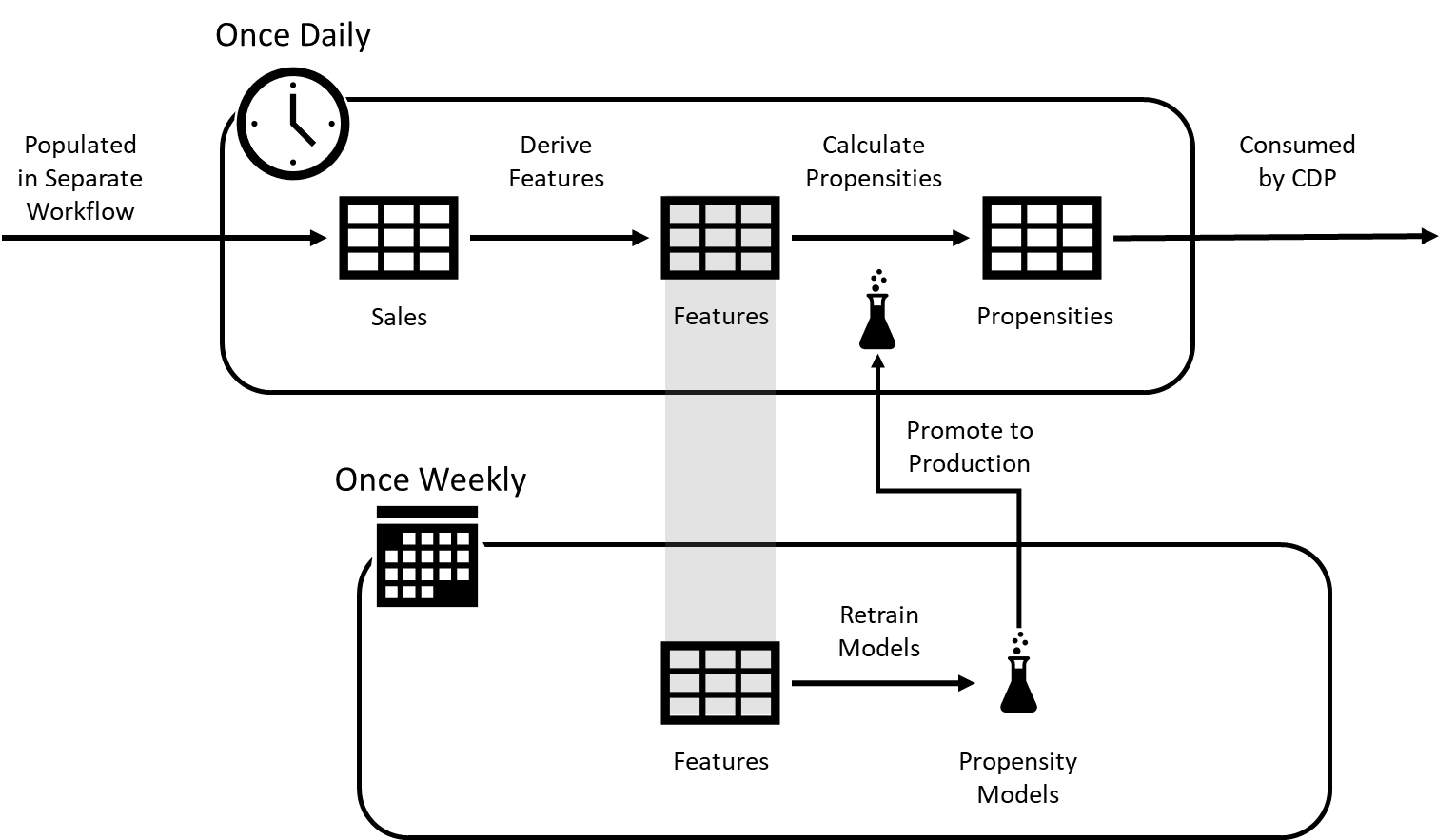
Within the first of the workflows (usually scheduled every day), the again workplace group focuses on the recalculation of options and scores. Info on lively product groupings is retrieved to regulate which options should be recalculated and these values are recorded to the Databricks function retailer.
The function retailer is a specialised functionality inside the Databricks platform that enables beforehand skilled fashions to retrieve the options on which they rely with minimal enter on the time of mannequin inference. Within the case of propensity scoring, simply present an identifier for the client and product group you want to rating and the mannequin will leverage the function retailer to retrieve the precise values it must return a prediction.
Within the second of the workflows (usually scheduled on a weekly or longer foundation), the info science group schedules every mannequin for periodic re-training. Newly skilled fashions are registered with the pre-integrated MLflow registry, which permits the Databricks atmosphere to trace a number of variations for every mannequin. Inner processes might be employed to check and consider newly skilled fashions with out concern that they could be uncovered to the scoring workflow till they’ve been totally vetted and blessed for manufacturing readiness. As soon as assigned this standing, the primary workflow sees the mannequin as the present lively mannequin and makes use of it for mannequin scoring with its subsequent cycle.
Whereas every workflow relies on the opposite, they every function on totally different frequencies. The function era and scoring workflow sometimes happens on a day by day or typically weekly foundation, relying on the wants of the group. The mannequin retraining workflow happens far much less steadily, probably on a weekly, month-to-month and even quarterly foundation. To coordinate these two, organizations can leverage the built-in Databricks Workflows functionality.
Databricks Workflows go far past easy course of scheduling. They can help you not solely outline the assorted duties that make up a workflow however the particular sources required to execute them. Monitoring and alerting capabilities show you how to handle these processes within the background, whereas state administration options show you how to not simply troubleshoot however restart failed jobs ought to they happen.
By approaching propensity scoring as two carefully associated streams of labor and leveraging the Databricks function retailer, workflows and the built-in MLflow mannequin registry, you may significantly scale back the complexity related to this work. Need to see these workflows in motion? Take a look at our Resolution Accelerators for Propensity Scoring the place we put these ideas and options into follow in opposition to a real-world dataset. We exhibit how configurable units of merchandise might be enlisted to be used within the growth of a number of propensity scoring fashions and the way these fashions can then be used to generate up-to-date scores, accessible to all kinds of selling platforms. We hope this useful resource helps retail organizations outline a sustainable course of for propensity scoring that can advance their preliminary personalization efforts.
[ad_2]