
[ad_1]
In a groundbreaking improvement, researchers from MIT’s Laptop Science and Synthetic Intelligence Laboratory (CSAIL) have launched a novel methodology leveraging synthetic intelligence (AI) brokers to automate the reason of intricate neural networks. As the dimensions and class of neural networks proceed to develop, explaining their conduct has change into a difficult puzzle. The MIT workforce goals to unravel this thriller by using AI fashions to experiment with different methods and articulate their interior workings.
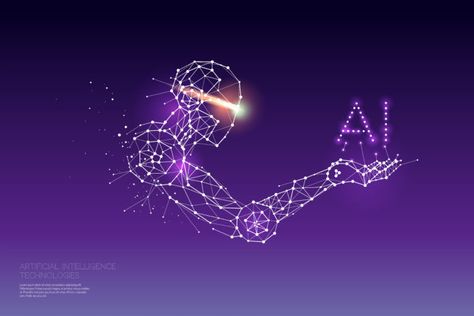
The Problem of Neural Community Interpretability
Understanding the conduct of educated neural networks poses a big problem, notably with the growing complexity of contemporary fashions. MIT researchers have taken a novel method to handle this problem. They may introduce AI brokers able to conducting experiments on numerous computational methods, starting from particular person neurons to whole fashions.
Brokers Constructed from Pretrained Language Fashions
On the core of the MIT workforce’s methodology are brokers constructed from pretrained language fashions. These brokers play a vital position in producing intuitive explanations of computations inside educated networks. In contrast to passive interpretability procedures that merely classify or summarize examples, the MIT-developed Synthetic Intelligence Brokers (AIAs) actively have interaction in speculation formation, experimental testing, and iterative studying. This dynamic participation permits them to refine their understanding of different methods in real-time.
Autonomous Speculation Technology and Testing
Sarah Schwettmann, Ph.D. ’21, co-lead writer of the paper on this groundbreaking work and a analysis scientist at CSAIL, emphasizes the autonomy of AIAs in speculation era and testing. The AIAs’ potential to autonomously probe different methods can unveil behaviors that may in any other case elude detection by scientists. Schwettmann highlights the exceptional functionality of language fashions. Moreover, they’re geared up with instruments for probing, designing, and executing experiments that improve interpretability.
FIND: Facilitating Interpretability via Novel Design
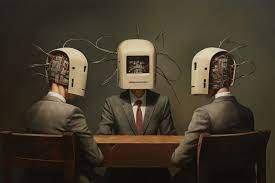
The MIT workforce’s FIND (Facilitating Interpretability via Novel Design) method introduces interpretability brokers able to planning and executing checks on computational methods. These brokers produce explanations in numerous types. This consists of language descriptions of a system’s capabilities and shortcomings and code that reproduces the system’s conduct. FIND represents a shift from conventional interpretability strategies, actively taking part in understanding advanced methods.
Actual-Time Studying and Experimental Design
The dynamic nature of FIND allows real-time studying and experimental design. The AIAs actively refine their comprehension of different methods via steady speculation testing and experimentation. This method enhances interpretability and surfaces behaviors that may in any other case stay unnoticed.
Our Say
The MIT researchers envision the FIND method’s pivotal position in interpretability analysis. It’s much like how clear benchmarks with ground-truth solutions have pushed developments in language fashions. The capability of AIAs to autonomously generate hypotheses and carry out experiments guarantees to deliver a brand new stage of understanding to the advanced world of neural networks. MIT’s FIND methodology propels the hunt for AI interpretability, unveiling neural community behaviors and advancing AI analysis considerably.
Associated
[ad_2]