
[ad_1]
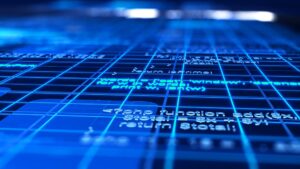
(Oselote/Shutterstock)
A startup known as Numbers Station is making use of the generative energy of pre-trained basis fashions comparable to GPT-4 to assist with information wrangling. The corporate, which is predicated on analysis performed on the Stanford AI Lab, has raised $17.5 million thus far, and says its AI-based copilot method is exhibiting a lot of promise for automating handbook information cleansing duties.
Regardless of enormous investments in the issue, information wrangling stays the bane of many information scientists’ and information engineers’ existences. On the one hand, having clear, well-ordered information is an absolute requisite for constructing machine studying and AI fashions which might be correct and freed from bias. Sadly, the character of the information wrangling drawback–and specifically the distinctiveness of every information set used for particular person AI initiatives–signifies that information scientists and information engineers typically spend the majority of their time manually getting ready the information to be used in coaching ML fashions.
This was a problem that Numbers Station co-founders Chris Aberger, Ines Chami, and Sen Wu have been trying to deal with whereas pursuing PhDs on the Stanford AI Lab. Led by their advisor (and future Numbers Station co-founder) Chris Re, the trio spent years working with conventional ML and AI fashions to deal with the persistent information wrangling hole.
Aberger, who’s Numbers Station’s CEO, defined what occurred subsequent in a latest interview with the enterprise capital agency Madrona.
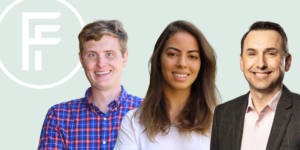
Numbers Station co-founders Chris Aberger and Ines Chami with Madrona Managing Director Tim Porter (left to proper) (Picture supply: Madrona)
“We got here collectively a few years in the past now and began enjoying with these basis fashions, and we made a considerably miserable commentary after hacking round with these fashions for a matter of weeks,” Aberger mentioned, in accordance with the transcript of the interview. “We shortly noticed that a variety of the work that we did in our PhDs was simply changed in a matter of weeks through the use of basis fashions.”
The invention “was considerably miserable from the standpoint of why did we spend half of a decade of our lives publishing these legacy ML methods on AI and information?” Aberger mentioned. “But additionally, actually thrilling as a result of we noticed this new know-how development of basis fashions coming, and we’re enthusiastic about taking that and making use of it to varied issues in analytics organizations.”
To be honest, the Stanford postgrads noticed the potential of huge language fashions (LLMs) for information wrangling earlier than everyone and his aunt began utilizing ChatGPT, which debuted six months in the past. They co-founded Numbers Station in 2021 to pursue the chance.
The important thing attribute that made basis fashions like GPT-3 helpful for information wrangling activity was their broad understanding of pure language and their functionality to offer helpful responses with out fine-tuning or coaching on particular information, so-called “one-shot” or “zero-shot” studying.
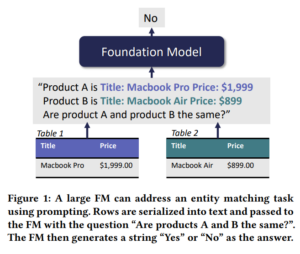
Picture supply: “Can Basis Fashions Wrangle Your Knowledge?”
With a lot of the core ML coaching performed, what remained for Numbers Station was devising a option to combine these basis fashions into the workflows of information wranglers. In response to Aberger, Chami wrote the majority of the seminal paper on utilizing basis fashions (FMs) for information wrangling activity, “Can Basis Fashions Wrangle Your Knowledge?” and served because the engineering result in develop Numbers Station’s first prototype.
One difficulty is that supply information is primarily tabular in nature, however FMs are largely created for unstructured information, comparable to phrases and pictures. Numbers Station addresses this by serializing the tabular information after which devising a collection of immediate templates to automate the precise duties required to feed the serialized information into the muse mannequin to get the specified response.
With zero coaching, Numbers Station was in a position to make use of this method to acquire “cheap high quality” outcomes on numerous information wrangling duties, together with information imputation, information matching, and error detection, Numbers Station researchers Laurel Orr and Avanika Narayan say in an October weblog publish. With 10 items of demonstration information, the accuracy will increase above 90% in lots of circumstances.
“These outcomes assist that FMs could be utilized to information wrangling duties, unlocking new alternatives to deliver state-of-the-art and automatic information wrangling to the self-service analytics world,” Orr and Narayan write.
The massive good thing about this method is that FMs can utilized by any information employee through their pure language interface, “with none customized pipeline code,” Orr and Narayan write. “Moreover, these fashions can be utilized out-of-the-box with restricted to no labeled information, decreasing time to worth by orders of magnitude in comparison with conventional AI options. Lastly, the identical mannequin can be utilized on all kinds of duties, assuaging the necessity to preserve complicated, hand-engineered pipelines.”
Chami, Re, Orr, and Narayan wrote the seminal paper on the usage of FMs in information wrangling, titled “Can Basis Fashions Wrangle Your Knowledge?” This analysis shaped the idea for Numbers Station’s first product, an information wrangling copilot dubbed the Knowledge Transformation Assistant.
The product makes use of publicly obtainable FMs–together with however not restricted to GPT-4–in addition to Numbers Station’s personal fashions, to automate the creation of information transformation pipelines. It additionally supplies a converter for turning pure language into SQL, dubbed SQL Transformation, in addition to AI Transformation and Document Matching capabilities.
In March, Madrona introduced it had taken a $12.5 million stake in Numbers Station in a Collection A spherical, including to a earlier $5 million seed spherical for the Menlo Park, California firm. Different traders embody Norwest Enterprise Companions, Manufacturing facility, and Jeff Hammerbach, a Cloudera co-founder.
Former Tableau CEO Mark Nelson, a strategic advisor to Madrona, has taken an curiosity within the agency. “Numbers Station is fixing a number of the greatest challenges which have existed within the information business for many years,” he mentioned in a March press launch. “Their platform and underlying AI know-how is ushering in a basic paradigm shift for the way forward for work on the trendy information stack.”
However information prep is simply the beginning. The corporate envisions constructing a complete platform to automate numerous elements of the information stack.
“It’s actually the place we’re spending our time at present, and the primary sort of workflows we need to automate with basis fashions,” Chami says within the Madrona interview, “however finally our imaginative and prescient is far larger than that, and we need to go up stack and automate increasingly of the analytics workflow.”
The corporate takes its identify from an artifact of intelligence and conflict. Beginning in WWI, intelligence officers arrange so-called “numbers stations” to ship info to their spies working in international nations through shortwave radio. The knowledge can be packaged as a collection of vocalized numbers and guarded with some sort of encoding. Numbers stations peaked in recognition through the Chilly Warfare and stay in use at present.
Associated Objects:
Evolution of Knowledge Wrangling Person Interfaces
Knowledge Prep Nonetheless Dominates Knowledge Scientists’ Time, Survey Finds
The Seven Sins of Knowledge Prep
[ad_2]